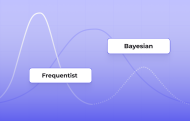
How to master continuous metrics with Kameleoon
Mastering continuous metrics in both Frequentist and Bayesian approaches can greatly boost the accuracy and reliability of your A/B tests.
By following best practices and understanding the strengths and limitations of each method, you’ll be better equipped to make informed decisions and drive stronger results for your business.
In this article, we explore how continuous metrics are handled with both Frequentist and Bayesian approaches on our Kameleoon platform. We break down the definitions, calculations, and interpretations of these metrics, and provide practical examples to show how they’re applied.
What are continuous metrics?
Continuous metrics are quantitative measures that can take any value within a range, like Revenue per Visit, Average Cart Value, or Time on Page.
Unlike binomial metrics, which have only two outcomes (like conversion or no conversion), continuous metrics offer more detailed insights into user behavior.
To help your team track and analyze continuous metrics, Kameleoon provides options for both Frequentist and Bayesian methods for more flexibility.
What is a Frequentist approach to continuous metrics?
Calculation and Interpretation
With a Frequentist approach, continuous metrics are analyzed using traditional methods like t-tests or ANOVA, relying on observed data to make inferences about the broader population.
Common Frequentist metrics include:
- Improvement rate. This is calculated as the difference in means between the test and control groups. It’s shown with a confidence interval, usually 95%, indicating the range where the true improvement rate is likely to fall.
- Reliability. A metric's reliability is determined by the p-value. If it’s below a set threshold (like 0.05), the result is considered statistically significant.
On Kameleoon, you can easily view these metrics and their associated statistical significance right in your test results dashboard, giving you all the information you need to make informed decisions.
Example of a Frequentist approach to continuous metrics in Kameleoon
Let’s say we’re comparing the Average Cart Value (ACV) between two versions of a landing page using Kameleoon:
Data.
- Control Group (Version A): [50, 55, 60, 65, 70]
- Test Group (Version B): [60, 65, 70, 75, 80]
Calculation.
- Mean (Control): (50 + 55 + 60 + 65 + 70) / 5 = 60
- Mean (Test): (60 + 65 + 70 + 75 + 80) / 5 = 70
- Improvement Rate: 70 - 60 = 10
Confidence interval. Kameleoon use a 95% confidence level to estimate the range where the true improvement rate is likely to fall. This interval shows the precision of our estimate and the degree of uncertainty. The exact calculation depends on the specific statistical analysis used, which isn’t detailed here.
Reliability. If the p-value is below 0.05, Kameleoon concludes that the difference is statistically significant.
Your team can view these results in the Kameleoon dashboard, giving you a clear and detailed look at the improvement rate and confidence intervals.
What is a Bayesian approach to continuous metrics?
Calculation and Interpretation
With a Bayesian approach, prior beliefs are updated with observed data to create a posterior distribution. This method is especially useful for continuous metrics, offering a more nuanced interpretation of the data.
Common Bayesian metrics include:
Improvement rate. This is the mean of the posterior distribution, representing the estimated average lift. Probability to win over reference. This shows the likelihood that the variation will outperform the control, based on the posterior distribution.
Credible interval. The bounds of credible interval indicate the range where the improvement rate is expected to fall, typically with 95% probability.
Kameleoon’s platform automatically calculates these Bayesian metrics, providing you with a detailed, probabilistic view of your test results.
Example of a Bayesian approach to continuous metrics in Kameleoon
Let’s continue with the ACV example from earlier, where we’re comparing the Average Cart Value between two landing page versions using Kameleoon. Now, we’ll apply a Bayesian approach with the Kameleoon platform.
Prior distribution. Assume a normal prior centered around zero with a standard deviation of 0.1.
Data.
- Control Group (Version A): [50, 55, 60, 65, 70]
- Test Group (Version B): [60, 65, 70, 75, 80]
Posterior calculation. Update the prior distribution with the observed data to obtain the posterior distribution. This step integrates prior beliefs with new data to refine our understanding.
Improvement rate. This is derived as the mean of the posterior distribution, providing an estimate of the average lift between test and control groups.
Probability to win. Represents the likelihood that the improvement rate is greater than zero, based on the posterior distribution.
Credible interval. Indicates the range where the improvement rate is likely to fall, typically with 95% probability. The exact bounds are calculated during analysis.
Kameleoon’s analytics engine handles all these calculations for you, so you can focus on interpreting the results instead of worrying about the computational details.
Comparison of Frequentist and Bayesian approaches to continuous metrics
Both Frequentist and Bayesian approaches come with their own advantages and limitations:
Frequentist approach
- Advantages: Simple, widely understood, and ideal for large sample sizes.
- Limitations: Less informative with small sample sizes and heavily dependent on p-values.
Bayesian approach
- Advantages: Uses prior knowledge, offers a more in-depth interpretation, and is great for small sample sizes.
- Limitations: Can be computationally demanding and requires careful choice of prior distributions.
Kameleoon supports both Frequentist and Bayesian approaches. This provides your team with the flexibility to choose which method best fits your needs and the ability to switch between them as needed.
Best practices for continuous metrics
Understand your data. Before choosing an approach, understand your data and the context of your analysis. Use both Frequentist and Bayesian approaches. Use both approaches to gain a more comprehensive understanding of your results.
Document your analysis. Keep detailed records of your methods and interpretations to ensure everything is transparent and reproducible.
Continuous metrics boost accuracy and reliability of your A/B tests
Mastering continuous metrics with both Frequentist and Bayesian approaches on Kameleoon can greatly boost the accuracy and reliability of your A/B tests. By following best practices and understanding the strengths and limits of each method, you’ll make smarter decisions and achieve better results for your business.
Learn more about how Kameleoon can help boost your experimentation program.