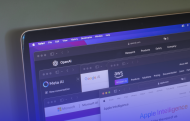
The practical playbook for AI in e-commerce
Microsoft, Amazon, and Google have funneled vast financial and computational resources into building AI models that offer endless opportunities for e-commerce businesses.
Using AI in e-commerce is fast becoming table stakes as business leaders recognize the opportunity to gain a competitive edge, capture market share, increase customer lifetime value, reduce operational costs, and improve conversion rates.
The challenge is determining which opportunities are worth pursuing.
A third of US online retailers claim to have fully implemented AI into business operations already. They deploy AI for tasks such as data analysis, A/B testing, promotions, personalization, customer service, merchandising, and fraud prevention. However, not all AI initiatives deliver positive results. That’s why the gap between those who harness AI effectively and those who deploy it without a clear strategy is widening.
To help teams determine where and how they should be using AI, Kameleoon and Conversion have created a practical playbook for using AI in e-commerce, which analyzes e-commerce use cases for AI across product, pricing, merchandising, experimentation, and operations. The report leverages Kameleoon's deep experience helping teams across marketing, product, and engineering scale experimentation using AI, personalization, and predictive targeting.
Below, we’ll briefly explore the e-commerce functions where AI can be applied. You can get the full analysis of all sixteen use cases by downloading the playbook.
- Product use cases: enhancing product presentation while saving you time
- Pricing use cases: optimizing profit with AI-driven pricing
- Merchandising & marketing use cases: answering every customer’s needs at scale
- Experimentation use cases: streamlining A/B testing workflows & team management
- Operations use cases: making e-commerce more secure and efficient
- Why download the practical AI playbook for e-commerce teams?
Product use cases: enhancing product presentation while saving you time
AI-generated product descriptions
Unclear product information drives abandonment, dissatisfaction, and returns. Generative AI can streamline content creation, producing high-quality descriptions in seconds. This is an ideal use case for businesses with large product catalogs or frequent product updates. What’s crucial, however, is enforcing human reviews.
User review moderation
Moderating reviews for fraud and inappropriate content is time-consuming—as is responding to them—but 89% of consumers prefer businesses that engage with all reviews. AI can take care of both tasks efficiently, and one survey found that 59% of consumers favored AI-generated responses over human ones. The challenge here is ensuring genuine reviews aren’t removed, which can damage brand trust.
Product review summaries
When making an online purchase, product reviews are the most influential factor for 65% of US consumers. But large volumes of reviews can overwhelm shoppers. AI-generated summaries solve this by extracting key themes from the reviews, helping customers make faster, more informed decisions. The critical factor for AI product summaries is that they are faithful to the sentiment and content of actual reviews.
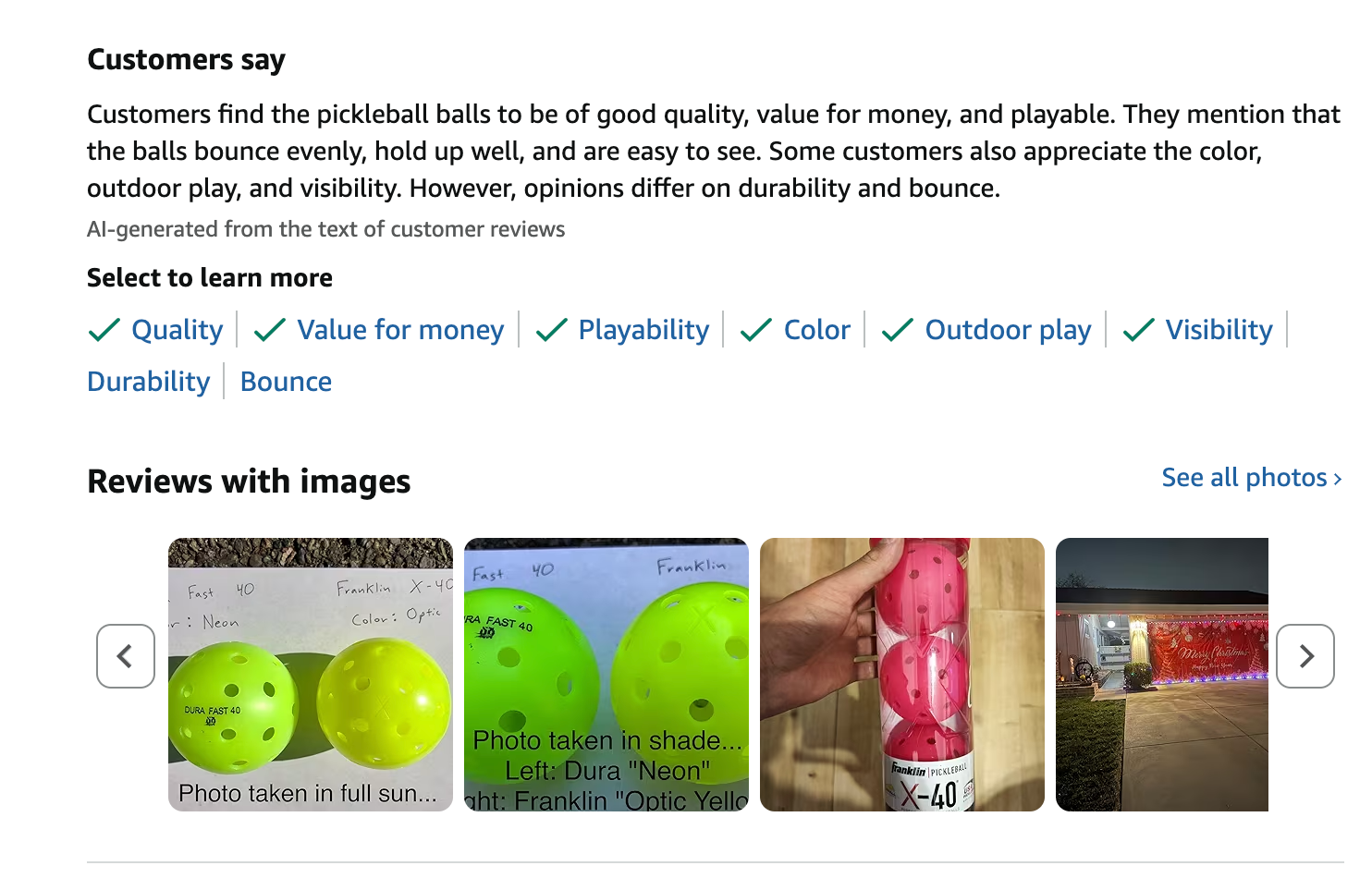
Virtual try-on technology
AI-driven virtual try-on technology lets customers preview products like clothing, footwear, eyewear, cosmetics, and home furnishings with enhanced realism and personalization. Studies show it can boost sales by 30% and cut return rates by 20%. While virtual try-ons can improve engagement, technical limitations or inaccuracies may lead to customer dissatisfaction if the virtual representation does not match the product.
New product development
AI can accelerate new product development by analyzing vast customer and market data, predicting trends, refining designs, generating mock-ups, and reducing time to market. In 2023, 62% of retail organizations allocated teams and budgets to integrate generative AI into future product and service development. But where do businesses stand regarding intellectual property and proprietary ideas generated by AI?
Product Images
Product images are crucial in purchasing decisions, with 75% of shoppers prioritizing visuals over other product details. Poor imagery contributes to higher return rates. While AI cannot generate accurate product images, it can speed up editing by creating backgrounds and ensuring visual consistency. Guidelines on using generative AI for internal teams are necessary if you use AI to help generate product images.
Get the full assessment of each product use case plus a comprehensive outline for implementation in the practical AI playbook for e-commerce teams.
Pricing use cases: optimizing profit with AI-driven pricing
Dynamic pricing
By leveraging AI for dynamic pricing, companies can increase profitability by up to 22%. Amazon reportedly makes 2.5 million price adjustments daily, adapting to demand and competitor activity. Dynamic pricing suits e-commerce businesses trading in fast-moving consumer goods (FMCG) and perishable items, where demand or shelf life can fluctuate rapidly. Price-sensitive markets and brands with extensive product lines and significant market data could also benefit.
But customer trust is critical here. While 70% of consumers accept dynamic pricing, transparency is essential to prevent negative reactions, and substantial regulatory compliance is needed to ensure pricing is fair.
Understand the key considerations when using AI for dynamic pricing in the practical AI playbook for e-commerce teams.
Merchandising & marketing use cases: answering every customer’s needs at scale
Website personalization
AI enables hyper-personalized marketing at every touchpoint, and 80% of shoppers expect tailored digital experiences. However, e-commerce leaders must balance relevance with privacy concerns. Good personalization delivers the right content at the right time, but to do so, businesses need solid customer data.
Marketing content
Generative AI can create content for ads, social media, and email campaigns. Half of marketers already use it for marketing tasks. While generative AI might take only seconds to produce content, time spent learning how to use the tools, refining prompts, and performing editorial reviews should be factored into any cost-benefit analysis.
Customer service chatbots
AI chatbots are now used by 77% of customer experience teams, with estimates suggesting conversational AI will cut contact center costs by $80 billion by 2026. While AI boosts response times, only 12% of customers prefer interacting with chatbots. That’s why e-commerce teams must consider a mixed-method approach to customer inquiries.
Find out how best to implement AI for each merchandising and marketing use case by downloading the practical AI playbook for e-commerce teams.
Experimentation use cases: streamlining A/B testing workflows & team management
Analyzing customer feedback and behaviour
E-commerce companies handle thousands of customer interactions daily, but extracting meaningful insights is challenging. AI can analyze large volumes of data to identify trends, pain points, and sentiment shifts, leading to improved products and services, and stronger brand loyalty.
This is a great way to use AI in e-commerce, particularly for businesses with strong test-and-learn cultures or brands that want to improve customer satisfaction scores. However, compliance with regulations such as GDPR and CCPA will be necessary, depending on the data and approach.
Meta-analysis of A/B tests
AI and machine learning can prepare data, aggregate thousands of experiments, and help predict outcomes. Doing this to perform a meta-analysis of previous A/B tests can help uncover macro-level trends and identify high-reward areas for testing. But to do this, you need to record your data accurately and have the right internal resources.
Identifying segmentation opportunities
AI can dynamically group customers based on real-time behavior, optimizing promotions and recommendations.
For example, Kameleoon created AI predictive targeting to identify visitors with a high likelihood of converting, which can then optimize the targeting strategy based on that propensity. Segmentation is also important after an A/B test concludes. A negative A/B test doesn’t mean it didn't work for some users. Teams who use AI to detect such opportunities find an average 15% uplift that would have otherwise been missed.
Experimentation workflows
AI can help teams with hypothesis generation, test prioritization, research questions, creative ideation, planning workshops, coding test variants, and writing design briefs.
From a management perspective, it can help tailor test report content based on the audience, summarize meetings, allocate resources, and even write a business case for additional investment. Ask AI to identify which stakeholders need to be involved in different tests and anticipate stakeholder feedback. The applications don’t stop there; AI can help with team management, too, from writing job descriptions and interview questions to supporting remote teams with advice using AI as a co-pilot.
Discover how to use AI to enhance your experimentation by downloading the practical AI playbook for e-commerce teams.
Operations use cases: making e-commerce more secure and efficient
Fraud detection
The e-commerce industry loses $48 billion annually due to online fraud—and it’s a growing problem. Machine learning can analyze real-time transactions, identify anomalies, and flag potential fraud. Effective AI-driven fraud detection enhances customer trust by safeguarding personal and financial information. However, false positives can lead to legitimate transactions being declined.
Inventory & supply chain optimization
AI and machine learning can analyze vast data sets in real time, identifying trade-offs across thousands of variables to optimize inventory, delivery, and supply chains beyond human capability. This improved forecasting can reduce costs by 15% and improve inventory levels by 35%.
However, integrating AI into existing supply chain systems can be complex, especially when companies use legacy systems. Full implementation can require changes to operation processes, machinery, warehousing, and significant change management to retrain staff.
Learn more about using AI in e-commerce operations in the practical AI playbook for e-commerce teams.
Why download the practical AI playbook for e-commerce teams?
The use cases highlighted above illustrate the breadth of applications for AI in e-commerce. This playbook contains a pragmatic overview of each, helping teams navigate the complexity and move beyond the hype to assess each opportunity. The playbook also contains:
- A decision framework to evaluate AI opportunities based on your business and circumstances.
- Guidelines on how to approach the implementation of AI for each use case.
- Key considerations, including ethical, technical, operational, and regulatory factors.
- Test ideas to help you validate AI effectiveness before full-scale deployment and optimization ideas once implemented.
Download the practical AI playbook for e-commerce teams and ensure AI becomes an effective addition to your e-commerce strategy.