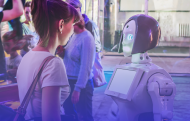
How do experimentation experts fit into an AI world?
Are you worried or excited about using AI in your work? Is it all hype, or can AI really add value? As with the arrival of any innovation, people tend to have polarized views—either it will steal our jobs, or it can be ignored, business as usual.
The reality is a lot more nuanced and less headline-worthy. While industry pundits suggest that AI will displace around 85 million jobs, they also predict that 97 million new jobs will be created. For teams to cement their value, they must learn to take advantage of AI technologies and refocus the time saved on more strategic or creative areas where AI cannot assist.
To understand how experimentation experts fit into this new AI world, we spoke to eight practitioners exploring new AI applications in their testing programs.
Your new co-pilot
Having spoken to many people across the industry, there’s no clear consensus on what tasks AI tools should be for. Opinions differ primarily around whether AI can help with data preparation and analysis. Johann Van Tonder explains how AI is already helping him with data analysis:
Analysis can now be done in a fraction of the time. I've done enough spot checks and cross-validation to know that the machine output is at least as accurate, if not more, than human counterparts. But what about hallucinations? It turns out, when you make side-by-side comparisons, humans hallucinate a lot more than we thought.
But others warn about wholly relying on AI as the datasets used often miss vital contextual information, as Ellie Hughes explains:
To expand on the type of context and human judgment still required, Florent Buisson provides an example:
What’s clear is that you should see AI analysis as a first pass. AI is a co-pilot and can help prepare and analyze data, but human overview is needed to sense-check the output.
Learn new skills to work with AI
The central theme emerging from our industry is that AI should be seen as an assistant to experimentation teams, not a replacement. However, to use this technology effectively, we might need to adapt our processes and learn new skills to get the most out of it. We’ve already written a guide to AI prompts to help you.
Eric Itzkowitz expands on the new skills experimentation teams need:
Given the need for these new skills, it’s worth adapting job descriptions to see if potential recruits have used AI tools. It’s a new technology, so don’t expect years of experience, but a general understanding and prior use of the tools is a good starting point.
With AI excelling in certain tasks, some existing CRO skills may decrease in demand. Jonathan Shuster explains what skills he thinks will be less in demand as well as AI features you might want in your testing tool:
AI’s CRO recommendations and analyses will surely improve over time, and we will need to embrace this inevitability. However, I have not yet seen any AI-driven technology that can effectively replace the organic ingenuity, intuition, collaborative spirit and capacity for consideration required to consistently deliver innovative and impactful experimentation.
Of course, my colleagues and I have a vested interest in avoiding obsolescence, so chalk up my perspective to self-preservation if you like. But I’m going with my gut at the moment. After all, I’m human.
To thrive in this new era of AI-supported experimentation, we should focus on how and where AI can be best applied to save us time so that we can add more value. Matt Beischel discuss this more:
To use a strained metaphor, Photoshop didn't replace designers; it increased a designer's efficiency by digitizing the editing process, replacing the time-consuming real-world physical work of compositing, cutting, pasting, airbrushing, etc.
CRO practitioners can get that same type of efficiency increase by using Large Language Model AIs across many of their regular tasks: generating research questions, market & competitor research, theme summarization, hypothesis generation/validation/standardization, ideation, content writing, etc. It's an excellent starting point, as long as you maintain editorial oversight of output.
Experts' roles as curators
A new type of AI application, synthetic audiences, has emerged. This is where a Generative AI creates an artificial audience modeled on existing user data. It can act and respond as real customers might. Initial claims suggest synthetic data could produce results 88% similar to typical audience insights.
Applications include using synthetic audiences in quantitative and qualitative research. Perhaps it could be used for A/B testing? Benjamin Skrainka answers this question:
The rise of causal Machine Learning (ML) tools is promising. These tools combine AI with causal inference techniques like double-debiased ML. This aids in estimating treatment effects, especially in quasi-experimental settings like marketing campaigns. However, A/B tests (or Randomized Controlled Trials) remain the gold standard for establishing causality.
AI can significantly enhance A/B testing by improving signal-to-noise ratios and identifying optimal treatment groups. But it cannot definitively prove causation without the randomized setup of an A/B test. Therefore, while AI is a valuable tool, it complements rather than replaces A/B testing in the quest for robust causal insights.
A critical new skill for teams is to know when and where to use AI and oversee its output. Whatever the use case for AI, it’s clear that experimentation teams will need to take on the role of curator. Part of this work will be to sense-check the underlying models used. Amrdeep Athwal explains why this is important:
Firstly, they tend to rely on large quantities of historical data to predict the present and future, and due to the constant state of flux, almost anything can change user behavior.
Secondly, there is also the matter of trust. Without knowing the accuracy of the predictions, we can't know how much trust to place in them and where they may be best used.
Finally, there is the issue of transparency. If a user inquires why they saw experience X, it would be useful to show the parameters that led to that experience. By testing these predictions, we can see some of the variables that led us to the prediction.
In essence, we can't treat AI predictive models like some infallible magical being that we must trust blindly; trust must be verified continuously.
We’ve already seen cases where predictive models have been used to show personalized pricing to customers, but a lack of oversight of the models meant the prices discriminated based on an individual's age. If teams choose to use such models, they must be able to understand and manage how they operate.
Data becomes even more important
As experimentation teams integrate more AI tools into their work, the focus on accurate, reliable data becomes even more important because this is what AI tools use to generate their output. Ellie Hughes explains why accurate data is crucial:
While getting accurate data has always been a challenging task, clean data is critical if you want AI to use it. As Ellie says, Garbage in, garbage out.
To ensure you have good data, you should focus on five aspects:
- Completeness of the data set.
- Relevance of the data to the questions you want to answer.
- Validity of the data. Is the data consistently recorded or in different formats?
- Timeliness of the data. Old data means conditions or user behavior might have changed since the data was recorded.
- Consistency across the data set. Do different systems or analytics tools show the same metrics?
Using AI to your advantage
AI tools are evolving daily. What’s considered the realm of imagination today might well be a reality tomorrow. No matter how advanced tools become, AI should be viewed as an assistant rather than a replacement.
While AI can handle tasks like data analysis and synthetically replicating audiences, human oversight remains critical, as does the need for accurate and reliable source data.
Instead of fearing AI will take over our jobs, we should focus on learning how to prompt AI to get the best output and adapting our processes to use AI as a time saver.
Ultimately, human judgment, intuition, context, and collaboration remain essential in achieving effective experimentation results, with AI acting as a valuable tool to complement, not replace, our expertise.
Thanks to the expert contributors for sharing their insights on this article.
- Ellie Hughes, Head of Consulting at Eclipse Group
- Johann Van Tonder, CEO at AWA Digital
- Florent Buisson, Applied Behavioral Economist
- Eric Itzkowitz, Director of Conversion Rate Optimization
- Jonathan Shuster, Digital Marketing Optimization Consultant
- Matt Beischel, CRO Consultant - Designer, Developer, and Optimizer at Corvus CRO
- Benjamin Skrainka, Lead Economist
- Amrdeep Athwal, Owner of Conversions Matter
If you want to set your new AI assistant to work, check out our guide to crafting AI prompts in experimentation.
Want to find out how AI could benefit your team's experimentation?