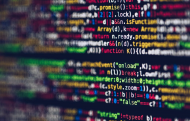
Understanding the statistical value of an A/B test
Developing a solid understanding of the statistical significance indicators of your A/B tests is essential to interpreting the results of your experiments successfully and to then improve your digital strategy. To this end, the confidence index provides precious information about your A/B tests - as long as you know how to interpret it.
1 What is an A/B test’s confidence index?
A confidence index relates to two versions (A and B) of a test and is obtained from the number of views (“visits”) and conversions for these two variations (so a total of four items of data).
The index is calculated by comparing the original version and the unique variation during an A/B test, but can also apply to a comparison of the B and D variations of an A/B/C/D test. Any A/B testing platform will provide this confidence index for each test. Even when one isn’t provided (on a web analytics solution, for example), it is possible to calculate it using a standard mathematical formula.
2 Cultivating a rigorous statistical approach to your A/B test results analysis
Users logically trust the statistical significance indicator provided by their testing solution. In the vast majority of cases, this is the correct approach. However, how you interpret the indicator can sometimes be wrong. For example, some users, lacking in-depth statistical knowledge may believe that it’s enough to observe how the conversion curves of the variations evolve. Others practically keep their eyes glued to the confidence index as they try to identify a trend in real-time.
You don’t need to become an expert in statistics or applied mathematics, especially as some concepts are counterintuitive. However, statistics has laws that cannot be ignored. Therefore, if you want a reliable result for your A/B tests, you need to know and understand some basic principles, as we’ll explain within this blog.
3 Distinguishing the increase in conversion and significance of the A/B test
The first thing to remember is that it’s impossible to predict the exact increase that a winning variation will provide. Or more exactly, you have no guarantee—in statistical terms—that the increase in conversion provided by the test is the “actual” increase in conversion, i.e. that which will actually be seen when in production.
The confidence index: the chance to beat the original
The confidence index represents the percentage of chances of obtaining the same result in the future—in strictly identical conditions (in terms of observations). Result means a single piece of binary information: either A beats B, or B beats A. This is why the confidence index is often called the “chance to beat original”.
What is the increase in conversions?
So, if you have a 15% increase in conversions for your variation and a statistical significance of 99%, this only means that your variation effectively has a 99% chance of outperforming the original. It doesn’t mean that there is a 99% chance that the increase in conversions will be 15% when the variant goes live on your website or the test is repeated. In fact, your actual increase in conversions could be any number, such as 3%.
How much importance should you assign to increases in conversion rates provided by your A/B tests?
This doesn’t mean that the increase in conversion rates generated by a test is totally meaningless, just that the confidence index doesn’t apply to it.
This is where the question of sample size comes in: with moderate traffic and conversion numbers, the standard deviation will potentially be very high. With a high traffic volume, it will be limited. In any event, this lack of a guarantee is not a problem. As soon as a variation beats the original, that’s the one that should go live on your website, even if the increase in conversions is minimal. Remember that actual conversion rates can rise as well as fall, so a 2% increase in conversions obtained in a test can turn into a 10% boost in conversions once that version goes live.
4 Watching the evolution of the confidence index over time makes no sense
The dashboards on some A/B testing solutions display a confidence index right from the beginning of the experiment. The problem with this is that the evolution of this indicator over time has zero value and can mislead inexperienced users.
A confidence index is obtained from a set number of observations and represents the percentage of chances that the same result will be obtained with the same number of observations in the future. So if you have an index of 90% that was only obtained over 50 visits, there is some probability that you will obtain the same result...but only over 50 visits.
Through extrapolation, you can then get three different significance indexes during a test:
- 90% at 1,000 visits
- 65% at 15,000 visits
- A final one of 95% at 50,000 visits
You shouldn’t take the first two values into account, since the last is the only one that is representative of your traffic when live.
From an applied maths point of view, a trend graph of the significance over time is meaningless. In reality, the confidence index should only be displayed once the test is over or close to concluding (i.e. has nearly reached the targeted number of visitors), to avoid the entirely natural temptation to regularly look at this figure.
This is why you should always run tests on all traffic and not just on a fraction of it. If it makes sense to start the test on a small portion of your traffic to make sure it is running correctly, then it is essential to extend this or you may draw false conclusions about the winning variation.
5 The confidence index doesn’t tell you when to stop a test
A confidence index should never be used as an indicator of when to stop a test. Unfortunately, the most natural reflex is to observe this index and to stop a test once it has exceeded a certain threshold, by convention, 95%. In reality, though, this has no statistical value. Usually, it is good practice to set a threshold of visits or conversions in advance, and only then to note whether or not the test is reliable.
To complete this first look at the statistical value of your tests, find out how to validate your experiments by using an A/A test, and make sure that your traffic volume is sufficient for successful testing.