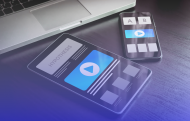
A/B testing vs. hypothesis testing: what’s the difference?
Optimization is a great approach to improving user experience and increasing conversion rates to drive business growth. A/B testing and hypothesis testing are two strong techniques brands can use to incorporate data-driven optimization for their processes.
These methods share similarities, but the terms cannot be used interchangeably. A hypothesis test is a statistical approach used to determine the likelihood that a particular hypothesis is true; A/B testing is often used to test hypotheses by comparing variants of a page or feature. This means that when you perform an A/B test, you are usually also performing a hypothesis test, but can hypothesis test without doing A/B testing.
What is A/B testing and hypothesis testing?
A/B testing is a particular type of experiment that compares two variants of a webpage, app, or feature to see which one performs better. Often, marketers, developers, and engineers will use A/B testing to test changes so they can understand how those changes will affect the user experience before committing to the variant or the original.
In order to run an A/B test, you have to:
- Create a hypothesis outlining how you think a particular change will impact an asset’s performance.
- Split your audience based on the hypothesis; usually this will be a random, 50/50 split.
- Run your test, showing each segment of the audience a different version of the asset.
- Analyze the results and implement the version that performed better.
Hypothesis testing is not a type of experiment, but rather an approach used to validate a theory about your product or service. It is broader in scope than A/B testing, and applies to many contexts beyond just product testing. It involves making an assumption and testing it using data to either confirm or reject it. Hypothesis testing assesses the effect of a change based on data analysis.
In order to run a hypothesis test, you have to:
- Establish null and alternative hypotheses.
- Determine a significance level, or p-value. This is usually 0.05, indicating a 5% chance of incorrectly rejecting the null hypothesis.
- Collect data, either from A/B tests or other experiments or collection platforms.
- Perform statistical analyses on the collected data.
- Reject or confirm the null hypothesis based on the p-value and analysis.
When to use A/B testing
A/B testing is ideal when you want to test a specific change in isolation. For example:
- Testing different headlines to see which one generates more clicks.
- Comparing two layouts to understand which one improves user engagement.
- Evaluating the impact of different calls to action (CTAs) on conversion rates.
You would use A/B testing when you’re comparing two distinct ideas to determine which one performs better.
When to use hypothesis testing
Hypothesis testing is valuable when you have a specific theory or assumption that you want or need to validate. A hypothesis test might, for example:
- Test whether a new feature improves user engagement compared to the existing one.
- Assess whether a promotional campaign leads to a (statistically significant) increase in conversions.
- Verify whether improving a website’s speed reduces its bounce rate.
A/B testing vs. hypothesis testing: key differences
As the examples above show, hypothesis testing is not limited to testing variants, but often an A/B test is a good way to prove or disprove a hypothesis. Here are a few more key differences between a/b testing and hypothesis testing:
1. Purpose and scope
- A/B testing focuses on testing changes to a website or app. It is a specific, short-term test run for comparatively quick results.
- Hypothesis testing is broader than A/B testing; it tests theories about your product or service without being limited to variations or to the short-term.
2. Type of question asked
- A/B testing asks “which version performs better?”
- Hypothesis testing asks “does this change have a statistically significant impact on the data set?”
3. Experimental design
- A/B testing is itself an experimental design: two or more variants with a clear outcome measure.
- Hypothesis testing often requires more complex designs and analyses, especially when testing multi-variable changes or campaigns.
Using A/B testing and hypothesis testing together
A/B testing and hypothesis testing are not the same thing, but they can be used together in your optimization efforts. You can use hypothesis testing to test wider theories about your product or website, then use A/B testing to validate specific changes or refinements based on the results.
For example, you could hypothesize that adding a certain feature to your product will increase user engagement. When the feature launches, you begin collecting data, suggesting that users are indeed spending more time using your product after the change than before. From here, you can form new hypotheses and apply A/B testing to further test which elements of the feature are driving that new engagement.
Remember—the key similarity in A/B testing vs. hypothesis testing is that both of them allow you to make smarter, data-backed decisions that can improve the performances of all your digital products. The idea of "A/B testing vs. hypothesis" is much more about knowing when to use each one, rather than which one is better.