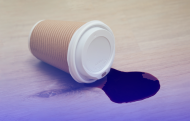
What are Type I and Type II errors?
Type I and Type II errors in hypothesis testing involve drawing inaccurate conclusions from experiments: Type I errors lead to false positive conclusions, where you incorrectly reject your null hypothesis when it's true, while Type II errors result in false negative conclusions, where you fail to reject your null hypothesis when it's false.
With both Type I and Type II errors, you draw wrong inferences, essentially seeing effects from your experiments when there are none and not seeing them when they exist. These errors happen when you fall for what your experimental data seems to be telling (though it’s not true).
Type I and Type II errors happen for various reasons across the experiment lifecycle, right from failing to plan a statistically significant or a sufficiently powered test and peeking at its results in the interim to simply happening to use poor sampling.
So, let’s see what Type I and Type II errors are, how they creep into your experiments and skew your findings, and finally, how you can avoid them.
What are Type I errors?
A Type I error is committed when you run an experiment and conclude that the change you tested impacted your target metric when, in reality, your change didn’t produce any significant effect. In other words, with Type I errors, you see an effect that’s not there and end up rejecting your null hypothesis or the default premise of your experiment that the change you’re testing won’t produce any effect when it’s true
Your probability of making a Type I error depends on your experiment’s significance level. Generally, a web experiment's significance level is set at 5% or 0.05, which means your chance of making a Type I error and rejecting a null hypothesis when it's true is 5%.
Understanding Type I errors
When you begin your experiment, you tell your experimentation solution your test’s significance level threshold (α). Your experimentation solution then computes and compares your test’s p-value (the probability of obtaining test results at least as extreme or more extreme as the results actually observed) with the significance level you set. If your test’s p-value is found to be lower than your significance level threshold (α), then you're looking at statistically significant results, and you can reject your null hypothesis safely and avoid making a Type I error.
A Type I error example in web experimentation
Suppose you’ve hypothesized a week-long A/B test on your homepage’s header messaging. You settled for a week as that would feed both the control and challenger an adequate sample size. Also, let’s say you’ve set the statistical significance level for this test to be the default 5%.
Assuming you started this test on Monday, you should wait until the coming Monday to see your result. But you checked your experimentation solution on Wednesday — peeked! — and found your test to be statistically significant with the challenger beating the control. Following your observation, you stopped the experiment, concluding that the null hypothesis was false and declaring version B the winner.
But post rolling out the change for all users, you end up with more or less the same numbers as before. The effect didn’t hold after all.
You committed a Type I error!
This example also shows how not peeking is one way to avoid making a Type I error. This is because the p-value for an experiment can change on a daily basis, often falling under the threshold in the interim before finally hitting its final value when the experiment runs its intended length.
What are Type II errors?
A Type II error is committed when you run an experiment and conclude that the change you tested didn’t impact your target metric when, in reality, your change did make a difference. In other words, with Type II errors, you miss the effect your experiment produces and fail to reject your null hypothesis (though it doesn’t hold).
Your probability of making a Type II error depends on your experiment’s statistical power. Generally, statistical power is set at 80% or 0.80, which means there’s an 80% chance that your experiment will be able to detect any actual effect that your experiment causes. This also means there’s only a 20% chance that you could miss the real impact of your experiment and make a Type II error.
Understanding Type II errors
Before you set up your experiment, you run a "power analysis" to determine the sample size you'll need to achieve your desired power level, significance level, and the expected effect size. Then, you input this sample size into your experimentation solution.
Once your experimentation solution delivers your experiment to the target sample sizes and it runs its intended length, you have a winner. Running an adequately powered test for its intended length is how you detect any true effects that your experiment causes and avoid failing to reject the null hypothesis, thereby committing a Type II error.
A Type II error example in web experimentation
Let's return to our header messaging A/B test example. Suppose this time, you had to show each version to 10,000 users for reliable results. But, because you didn’t see a winning version immediately, you concluded your test early (without showing it to enough users and meeting your sample size requirements).
But then, maybe you interviewed the customers you acquired during the experiment and asked them about what prompted them to convert, and they underlined how the messaging spoke to them. Using these learnings, you decide to rerun this test, but this time, you let it run its intended length and let both versions reach their target user populations. And this time, you saw that the challenger beat the control.
So, you had initially committed a Type II error in this experiment.
How are Type I and Type II errors different?
When you make a Type I error, you reject a null hypothesis even though it's true, whereas when you commit a Type II error, you fail to reject the null hypothesis when it should be rejected. In terms of web experimentation, with Type I errors, you detect effects that don’t exist. In contrast, with type two errors, you fail to detect existing effects.
With Type I error, you’ll end up rolling out a version you believe – and have erroneously concluded through your experiment – to impact your target metric positively. At best, such a change won’t impact your numbers (as the lift you saw won’t hold). But you can end up hurting your conversions, too, when you roll it out to your entire user base. On the other hand, with a Type II error, you’ll end up sticking to the status quo, and any improvement that the experiment would have made (if you hadn’t erroneously ended it by making a Type II error) is lost. Both can prove costly in their own ways.
Type 1 errors are easier to detect as the “uplift” you saw didn't sustain. Another way to detect a Type I error is to see a negative impact on your target metric with the full rollout. In contrast, Type II errors are more difficult to detect as you conclude the challenger was no better than the control and stick to the default.
How to approach and minimize Type I and Type II errors
Reducing the likelihood of one type of error makes you more vulnerable to making the other. If you reduce the value of your significance level threshold, you lower your chance of making a Type I error. So if instead of setting alpha to 5%, you set it to 1%, you’ll be 99% sure that the results you’re seeing from an experiment are there for real and not by chance. However, reducing alpha reduces a test’s power, making you more vulnerable to a Type II error. A test that’s not sufficiently powered will not be sensitive enough to detect differences when they're there. Lowering alpha means while you’ll likely not detect a non-existent effect, you might as well simply miss it when it’s there.
Get your test logistics right. Go for a 5% statistical significance level threshold and 80% power. There’s nothing magical about these numbers, but they’re the standard for web optimization. Also, determine things like your sample size before you set up your experiment; otherwise, you can get prone to manipulating metrics like the detectable difference just to run conclusive experiments.
A good sample size is your best defense against both Type 1 and Type 2 errors. But this isn’t without its challenges. A considerable sample size means your test takes more time to run, which impacts your testing velocity. For low-traffic sites, this means really long-running experiments.
A good experimentation solution does its bit. Invest in a good experimentation solution that comes with an accurate stats engine. Investigate the different statistical calculations it uses, for example, for computing the p-value.
Add A/A tests to your experimentation mix.
A/A tests can detect issues with your experimentation solution and help iron out many issues that can lead to Type I and Type II errors.
The bottom line on Type I and Type II errors
While you can't eliminate Type 1 and Type II errors, you can significantly lower their chances.<
And doing so is essential as routinely running into Type I and Type II errors can derail your optimization program. Repeatedly not seeing the results of your experiment produce sustainable growth (because of Type I errors) or failing to prove what you correctly hypothesized (because of Type II errors) can make you question your entire optimization process. Both errors have their costs and consequences. In web optimization, depending on what you’re testing, you can be more accepting of either of the errors.
One middle ground, though, is a more balanced approach, where you set a good significance level, run an adequately powered test, and let your experiments run their intended length. And remember that a big sample size protects you against both Type I and Type II errors.
Check out our chat with Ronny Kohavi for more on how to avoid these inevitable hypothesis-testing errors.