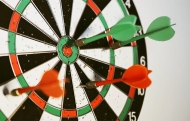
How to avoid common data accuracy pitfalls in A/B testing
With access to the right tools, anyone can run experiments. But not everyone can run trustworthy experiments.
There are several testing pitfalls that can create dramatic data inaccuracies, negatively impacting test trustworthiness.
Because your A/B test results are only as good as the data behind them, it’s important to avoid trustworthiness traps.
Otherwise, you risk making costly money-losing mistakes that jeopardize revenue and your reputation.
With diminished trust in your abilities, management may lose confidence in your or your team’s work.
With lost credibility, organizational buy-in becomes that much more challenging, threatening the overall feasibility of your testing program.
It’s a vicious downward spiral.
To prevent these negative consequences, it's important to avoid common testing pitfalls that lead to inaccurate, unreliable, untrustworthy data.
To provide tangible solutions on how to overcome key data inaccuracy pitfalls, we conducted an interview with top test trustworthiness expert, Ronny Kohavi.
Ronny is the former Vice-President and Technical Fellow at Microsoft and Airbnb. Over his 20+ year experimentation career, he’s run thousands of online experiments and has assembled his observations into a best-selling book, Trustworthy Online Controlled Experiments: A Practical Guide to A/B Testing. He’s also published dozens of papers on the topic, and offers a must-take course on accelerating innovation with A/B testing.
In this webinar, Ronny shares several statistical solutions you can use to avoid common data accuracy pitfalls and obtain trustworthy test results.
Adding a practical practitioner's perspective, Deborah O’Malley, founder of GuessTheTest and ConvertExperts, suggests several considerations to move beyond data inaccuracies, especially for agencies and lower traffic sites.
Together, they outline the 7 most important test trustworthiness pitfalls and how to avoid them. Access the webinar replay here.
Main takeaway: running a test is simple. Running a trustworthy test that yields accurate, reliable results is much more complex. To get trustworthy data, there are 7 main data inaccuracy pitfalls to avoid.
Pitfall #1. Interpreting p-values incorrectly
In A/B testing, statistical significance is a metric used to determine whether a test result is trustworthy and, therefore, a true winner.
Statistical significance is evaluated based on something called a p-value.
To ensure trustworthy test results, you need to know how to properly interpret p-values. The problem is, p-value is a highly misunderstood concept.
To understand p-value, it is helpful to begin by exploring the concept of the scientific method.
The scientific method is a process. It involves making an observation, forming a hypothesis, conducting an experiment, and analyzing the results.
A basic assumption of the scientific method is that the current known state holds true.
In A/B testing, this outlook assumes that, until proven otherwise, there is no conversion difference between variations.
A stance known as the null hypothesis.
Assuming no difference between variations, p-value determines the probability of actually observing a difference between variations.
If the probability of observing a difference is less than or equal to 5% (p ≤0.05), you reject the null hypothesis and say there is a difference. This difference is unexpected, so it’s a noteworthy, significant finding; therefore, the result is statistically significant.
That’s all p-value tells.
A very common mis-understanding is that, based on a p-value of 0.05, you can be 95% confident the treatment is different. However, that interpretation is incorrect.
You can’t know for certain because there’s always the risk of a false positive.
False positive risk
A false positive occurs when a conversion difference appears — even though there really isn’t one.
As this chart shows, even at some of the world’s largest and most advanced testing organizations, the false positive risk, also known as a False Probability Risk (FPR), may be as high as 26.4%:
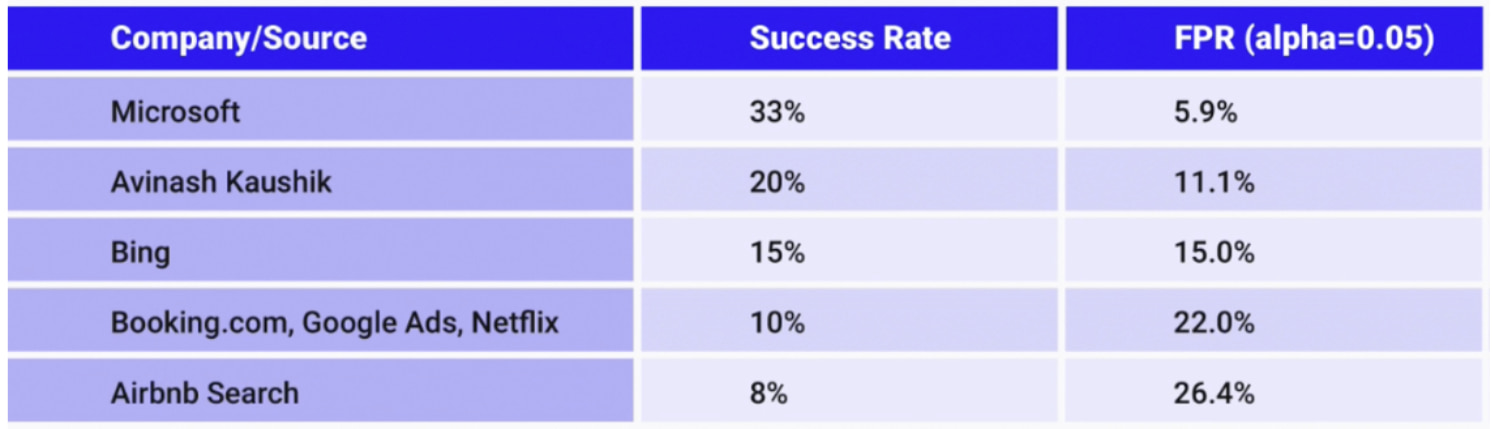
Therefore, in your own testing practice, before you can truly declare a winning test, it’s important you consider the FPR.
To calculate the FPR, you should assess the history of your own test successes or use the above industry success rate chart.
Additionally, one of the best ways to reduce the risk of false positives is to ensure your study has adequate statistical power.
Pitfall #2. Running tests with insufficient statistical power
In A/B testing, power measures the percentage of time a real conversion difference will be detected, assuming one truly exists.
The standard level of power is 0.8 (80%).
This amount directly ties into sample size requirements. As a general rule of thumb, a properly-powered test needs at least 121,600 visitors per variation.
If you’re running tests with dramatically smaller samples, beware!
You may still get statistically significant results. But, as explained by Georgi Georgiev, the results are not to be trusted. Studies with small samples are likely underpowered, and any lift detected is often a gross exaggeration.
Experiments with low statistical power can lead you to fall into the trap of the “winner’s curse” in which the statistically significant result looks like a win to be celebrated. But, in fact, the so-called win is actually a massive exaggeration.
If tests are implemented on these erroneous results, the end outcome may be a curse more than a cause for celebration.
Pitfall #3: Overlooking Sample Ratio Mismatch (SRM)
However, even if your experiments are adequately powered, if you’re not checking for Sample Ratio Mismatch (SRM), the results can still be faulty – and are not to be trusted.
SRM occurs when traffic is unintentionally unevenly split.
In a typical split test, the ratio of traffic between control and treatment should be roughly equal. If one variation receives notably more traffic than the other, there’s a SRM issue.
Even a 0.2% difference in traffic allocation can create SRM. Although a seemingly small amount, it’s enough to skew results – which means findings shouldn’t be trusted.
SRM is a common occurrence. In fact, based on a p-value of <0.001, 6% of all tests may end up with an SRM issue.
There are more than 40 reasons why SRM might occur.
Most relate to redirect issues, test code problems, or randomization bugs with specific traffic segments. For example, users coming from an ad may always get assigned one variation. Or one version may be exposed to heavy bot traffic.
To ensure your tests don’t have SRM, you need to diagnose and course-correct for it. This paper provides a great starting point on how to do so.
You should also choose an enterprise-grade testing tool that has SRM detection built in to it.
Kameleoon offers a built-in SRM checker. Through in-app notifications, you’ll get real-time alerts anytime a SRM is suspected or detected. It’s like having a seatbelt light in the car: automated and convenient so you never need to worry about an undetected SRM issue.
Pitfall #4: Failing to run A/A tests
One of the best ways to detect SRM, and spot other outliers in your tests, is through a diagnostic technique known as A/A testing.
In A/A testing, traffic is equally split, but both the control and treatment are exactly the same.
Through this set-up, you’re looking to confirm that there is no difference between variations. If this outcome occurs, it shows things are working correctly.
However, if you find a statistically significant difference between results, it indicates a problem.
Like SRM issues, you may have bugs, tracking, or reporting issues. That said, about 5% of all A/A tests will yield a false positive. So take note of this number before panicking.
Although A/A tests have been criticized for being traffic and resource-intensive — because they can distract from running “real” tests that bring in conversions -- they shouldn’t be overlooked. They’re an important diagnostic tool to confirm trustworthy test results.
In fact, A/A tests don’t have to take resources away from your A/B testing program. You can just as effectively run A/A tests offline.
To do so, look at recent test results, split and compute the metrics, and see how many results come out statistically significant. Offline A/A tests aren’t expensive to run, don’t reduce your testing bandwidth, and are still very effective at identifying issues.
To help you identify bugs, and ensure your test results are totally trustworthy, you should be continually running A/A tests either on or offline.
Pitfall #5: Disregarding Twyman’s Law
If you see test results that look unusual or surprising, take note.
A principle known as Twyman’s Law states that any figure that looks interesting or different is usually wrong — and not to be trusted!
In testing, Twyman’s law can play out in many ways.
For example, if you have a mandatory birthday field on your form, you might have an unexpectedly high number of users with the birthday 01/01/01.
An interesting finding, you may question whether so many users were really all born the same day. The answer is: probably not! More likely, users were just lazy and ignored the pre-filled date dropdowns.
With Twyman’s Law, if you see strange data trends, or other massive conversion improvements, be skeptical. Triple check the results before you celebrate.
Otherwise, you may end up falling victim to the winner’s curse.
Pitfall #6: Failing to adjust testing techniques for data disruption
Yet, while test trustworthiness issues can be problematic, perhaps the greatest trap of all is failing to adjust to the changing testing landscape.
The emergence of AI-related tools, increasing browser privacy restrictions, and shifting consumer patterns are all bringing changes to how we collect and interpret data.
To maintain trustworthy tests, experimenters need to be aware of these impending changes, adjust to them, and adapt their testing frameworks.
Not adopting contextual bandits for traffic allocation
One easy to overlook aspect is the increasing adoption of AI-related tools.
Experimenters who fail to adopt progressive AI and machine learning frameworks risk getting left behind.
For example, contextual bandits are an increasingly popular experimentation technique. They use a machine learning framework to test different outcomes and determine which is the most rewarding.
Applying contextual bandits to experimentation holds great promise; it means optimizers can quickly find the best performing variants with little effort.
Not understanding the implications of browser privacy restrictions
Increasingly stringent browser privacy protections, restrictions on third-party and first-party cookie collection, and Apple’s Intelligent Tracking Prevention (ITP) all limit the ability to accurately track and collect user data.
However, a technique known as server-side tagging is emerging as a viable way to continue data collection for experimentation.
Kameleoon is, currently, one of the few vendors that has proactively created a server-side solution. It’s a short snippet that can simply be installed through an HTTP header. The snippet works on all browsers, even if cookies have been removed, is both GDPR- and CCPA- compliant, and won’t slow your website.
To ensure continued test trustworthiness, experimenters should select a vendor that has a server-side solution already in place.
Failing to factor in attribution windows
An attribution window can be thought of as the length of time conversion data is collected during a test.
Most experimentation platforms only account for conversions while the test is running.
But this data collection method is problematic.
It may not be well-matched with your organization’s unique sales cycle, lead nurturing sequence, seasonal trends, churn rates, or other business factors.
For example, let’s say you’re an e-commerce retailer and sell shoes for hundreds of dollars. Imagine you run a five-week test using a standard attribution window. During this time, the data shows you achieved a 2.5% conversion rate.
But, by setting your custom attribution window, what you find out is the conversion rate is actually 2.8%.
This difference appears small, but amounts to a 12% change – which may equate to thousands of dollars in unaccounted revenue.
The end result is conversion data you can’t totally trust.
To overcome this issue, Kameleoon has created a fully customizable A/B testing custom attribution window. Through the custom attribution window, you can easily select the amount of time you’d like to monitor conversion for every experiment.
This solution reduces the likelihood of faulty, incomplete data sets – helping improve test trustworthiness.
Pitfall #7: Neglecting other pitfalls like “peeking” and bots
While these top testing pitfalls can greatly reduce the accuracy of data and trustworthiness of test results, they’re not the only issues you need to look out for.
Other common test trustworthiness traps include:
- Peeking: Stopping the test early, or ‘peeking’, at the test results before sample size requirements have been met.
- Failing to align the conversion objective, also known as the Overall Evaluation Criteria (OEC), with what’s being tested. For example, measuring an individual metric, like Revenue Per Visitor (RPV), when conversions are measured by collective page visits.
- Getting hung-up on small data discrepancies between third-party platforms and not picking a single source of truth or looking for overall data patterns.
- Computing confidence intervals incorrectly.
- Neglecting bots which make up a huge amount of traffic and can impact SRM.
- Overlooking instrumentation issues and outliers.
Failing to overcome these testing issues can be deeply detrimental to the trustworthiness of your test data and impact the reputation and credibility of your experimentation program.
Avoiding all test trustworthiness traps
There are many test trustworthiness traps experimenters can easily fall into.
However, there are also several smart solutions you can apply to avoid getting duped by data inaccuracies.
By being aware of, and attempting to overcome these testing pitfalls, you’re more likely to run accurate, reliable experiments.
With data you can trust, you can confidently make decisions that increase your revenue and build your testing program’s reputation and credibility.
For more on how to achieve trustworthy online experiments, learn how to overcome the three biggest challenges with data accuracy.
To learn more about how to run trustworthy tests through Kameleoon, request a demo.