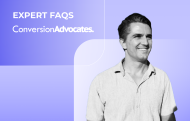
How can AI support experimentation?
This interview is part of Kameleoon's Expert FAQs series, where we interview leaders in data-driven CX optimization and experimentation. Bradley Rodé is the Lead Data Scientist at ConversionAdvocates. He is passionate about finding new ways to leverage data science techniques to help organizations grow.
How can AI assist with A/B testing?
AI can assist with analyzing test results and formulating new hypotheses. You can input experiment data, or any other tabular data, into a large language model and have the model accurately write up an analysis of the experiment findings, create visualizations, study segments, and perform any additional calculations you ask. Large language models can now even run Python code inside the chat user interface, speeding up any advanced analysis techniques by having the AI write and execute the code all by itself.
Now that the AI has studied the performance of your experiment, you could certainly ask it for hypotheses to test next. Feeding it additional data from past experiments or other data sources can help improve the hypothesis generation even further. That said, the jury is still out on how effective AI is a hypothesis generation over human beings. The AI’s predictions will always be beholden to the data it was trained on.
On the other hand, experimentation professionals can consider many things that AI does not. Such as brainstorming sessions with internal and external stakeholders, from qualitative aspects of user research such as body language or tacit knowledge.
Can AI help with estimating causal effects?
Randomized control trials are the gold standard for estimating causal effects. But, there are additional things you can do to assess the impact of elements on your website using existing web analytics data.
These techniques are often used in biostatistics and econometrics but rarely discussed in product analytics. One method is called “matching,” where each user that triggers “an event” gets matched to an identical user that did not trigger the event. This creates two very balanced datasets ready for analysis, equal in their distributions of confounding variables and differing only in the event we are studying. The more we can rule out confounders in this fashion, the closer we can estimate a causal effect. Machine learning can help in this process as well.
One step in the casual inference workflow is assigning a “probability of treatment,” where machine learning algorithms can excel over traditional statistical models in calculating the probability. AI advances are also accelerating our estimation of causal effects from observational data. It will never replace the need for good quality randomized controlled trials, but it can provide us with a more thorough understanding of how different components are impacting our users’ probability of converting before forming any hypotheses to test.
What areas of user research and data analysis can be enhanced with AI?
AI is extremely good at synthesizing large amounts of data, which includes written text.
One impactful use case is employing AI to analyze and classify customer reviews by topics and emotions. This can lead to surprising insights, as I recently found when working with a clothing company that positioned itself as high-end and committed to sustainability.
Using AI to analyze thousands of customer reviews, we found that positive reviews almost never mentioned sustainability, and negative reviews almost never mentioned the price. It was the fit and quality of the material that customers cared about the most, whether the customer left a one-star or five-star score. Insights like this can lead to new experiment ideas focused on what customers actually care about and not what we assume they care about.
How can AI help to optimize products and personalization?
Using AI to optimize digital products has been a flourishing field for many years now. Take personalization, which is essential for websites with a wide variety of products. As the product range grows, so does the diversity of the user base and the number of potential permutations for personalization. AI can help generate tailored recommendations and a unique user experience to meet the needs of each diverse customer.
Other popular ways of using AI to create personalized experiences are;
- Recommender systems for suggesting products to buy or movies to watch.
- Cluster analysis to group similar users and show them different experiences, products, or services.
- Churn prediction to target users about to leave the site or checkout.
What area of experimentation will be impacted most by AI in the future?
For me, the most significant impact will be on how we identify patterns to create winning variants.
It’s easy to imagine a future where artificial intelligence collects data on everything to do with experiment variants—copywriting changes, CTA changes, design changes, code changes, plus all of the result data. AI could start (very accurately) predicting your next winning test and identifying any patterns behind these winning variants.
In addition, AI is becoming so good at design and coding that in the near future, it may be able to code your next winning variant and launch the test all by itself.
Bradley, you started your career as a helicopter search and rescue professional. What led you to switch career paths, and do you have any advice for others looking to make a similar career move into data science?
Although seemingly quite different, search and rescue and data science both involve problem-solving, something I have always enjoyed doing as part of my job.
Working in search and rescue, you must be quick, decisive, and effective under pressure. The only way to achieve this is through constant preparation and training. Training involves thinking through every possible scenario ahead of time, calculating the best courses of action, and practicing these scenarios repeatedly.
Data science also involves evaluating many potential solutions to a problem, choosing the best course of action, and constantly learning new things to keep your skills sharp. Although it is certainly less of an adrenaline rush, working with data is a thrilling field that challenges and excites me every day.
My advice for those thinking of making career changes is to follow your passion. I got into search and rescue after joining the military as my first job out of college and was immediately hooked.
After leaving the military, I had a similar feeling of excitement when first learning about prediction models during a statistics course in business school. In both scenarios, what I was learning didn’t feel like work, so I stuck with it, trying to get better each day. When you truly enjoy what you’re doing for its own sake and not for some future reward, it’s a great sign that this is a career for you. So, for anyone passionate about working with data, just keep following your passion, getting better at it each day, and eventually, the career will come.