
Dynamic traffic allocation: optimize your A/B tests
Dynamic traffic allocation allows you to optimize your A/B tests by distributing the traffic on your website to the most efficient variations before the test phase has even finished. This post explains how this technique works and the benefits it delivers if you follow best practice.
Dynamic traffic allocation is sometimes presented as a revolutionary alternative to A/B testing, or even as a competitor to predictive targeting. The reality is more straightforward: it is an optimized testing approach which does not address the same issues as “classic” A/B testing.
1 The multi-armed bandit problem
Dynamic traffic allocation can resolve what is known as the multi-armed bandit (MAB) problem. This is a mathematical problem posed in probability theory, with the name coming from the nickname of old-fashioned slot machines with an arm on the side that you pull. It is easier to show by giving an example.
Imagine that you are in a casino, looking at several slot machines. Some machines distribute big payouts, others smaller ones. How do you choose which machines to play on in order to maximize your winnings?
There are two main approaches:
- Quickly test some of the machines, identify one that pays out a lot, and decide to stay with that machine: this is exploitation.
- Continue testing all the machines, one by one, hoping that the next one will let you win more: this is exploration.
What solution should you choose between exploitation and exploration? Multi-armed bandit algorithms can resolve this problem by simultaneously combining a test period and a winning period, i.e. exploiting and exploring at the same time.
What strategy should you use to optimize your website?
Let’s change the example to optimizing your website. You want to integrate a new element and you have two possible options:
- Continue using an existing element that works well with your visitors
- Choosing a new element and A/B testing its efficiency in the hope of obtaining results that are better than your current practice.
You are going to have to choose between exploitation and exploration approaches.
If you choose to exploit the resources whose performance you know, you’ll get adequate results but you won’t know if alternatives work better.
If you base your approach on exploration, then you’re taking the risk of testing an element that could be unprofitable, but at the same time could also surpass all your other results.
How A/B testing addresses the multi-armed bandit problem
A/B testing compares different versions of an element on your website with the aim of discovering which is the most efficient with your visitors. This technique allows marketing teams to make informed decisions so as to optimize the website user experience.
However, with A/B tests, you need to implement a strict process and learning period to gather data before deciding to make changes.
So A/B testing lets you analyze (exploration) the performance of different elements on your website, but you have to wait for the end of the test before you can draw any conclusions from it and put these into action (exploitation).
This is where dynamic traffic allocation comes into play, aiming to try to combine exploration and exploitation.
2 What is dynamic traffic allocation?
It’s a simple concept: dynamic traffic allocation can modify the distribution of traffic based on the first results observed during the test period. A multi-armed bandit algorithm progressively redirects visitors towards the variation that works best.
So dynamic traffic allocation lets you exploit your results while continuing to carry out tests.
Let’s take the example of a brand that would like to determine what type of CTA converts most efficiently on its website. In a traditional test, the traffic is distributed equally between each variation until the end of the test. So, if two types of CTA are tested, 50% of the visitors will be exposed to variation A, and the rest to variation B.
With dynamic traffic allocation, the distribution can change depending on the performance of the element being tested. If variation B shows a high conversion rate, then the traffic distribution can be adjusted accordingly.
3 Why should you use dynamic traffic allocation?
To maximize conversions
Thanks to dynamic traffic allocation, visitors are directed towards the variation that works best. Consequently, you minimize the losses due to A/B testing while continuing to explore new possibilities.
To minimize risk
Conversely, you minimize risk when you test new elements since, if they don’t work, the traffic allocated to this variation will be gradually reduced.
4 When should you use bandit tests instead of A/B tests?
Multi-armed bandit algorithms are useful as they minimize the cost of lost opportunities during the exploratory phase of an A/B test by combining exploration and exploitation.
When time is limited
Dynamic traffic allocation is very useful when the exploration period and the exploitation period have to take place over a short time. This is particularly the case when you test headings for articles or for promotional campaigns with a limited timespan (such as holidays, events).
If you don’t have much time to analyze results
Dynamic traffic allocation can also be useful when you want to run tests without having to closely follow their progress and results. By putting the bandit test in place, the learning time will be longer but the traffic will be directed towards the best variation without ending the exploratory period.
5 Is dynamic traffic allocation suitable for all websites?
If this practice can combine the exploration period and the exploitation period, then why not make it more widespread?
Website traffic
With the pure exploration period reduced, your website needs to have high traffic in order to obtain significant results. Indeed, if you only have very few visitors on your website, the first results obtained will not represent the general trend and you run the risk of drawing bad conclusions from them.
Significant results
The multi-armed bandit technique takes more time than an A/B test to obtain significant statistical results since the algorithms distribute the traffic according to the results obtained. This means many more visitors need to be tested to get conclusive results.
If the test isn’t performed for long enough, you run the risk of obtaining false results. For example, if a variation is tested right at the start of the test and is initially declared a losing one because traffic is not yet large enough, then more time will be needed to understand its true benefits.
Loss of conversionS over the long term
You can use multi-armed bandit tests to continuously optimize your website, but in this case you will miss out on a certain number of conversions in the long term.
In fact, with this technique, you’ll need much more time to obtain significant results and, during this period, a part of your traffic will always be directed towards the least efficient variations.
In order to maximize conversions in the long term, the best solution is to quickly obtain a winning variation with an A/B test and then to implement it on your website.
6 Can you personalize with multi-armed bandit algorithms?
With a classic bandit test, the version displayed to visitors is only based on the results obtained and ignores the context.
More evolved versions of the algorithm exists: contextual bandits, extension of bandit tests, or simplified versions of reinforcement learning.
Contextual bandit algorithms are capable of taking into account the context (visitor journey, origin, geolocation, etc.) to refine the traffic allocation: if an initial visitor converts on the variation ‘x’, then other visitors with a similar profile will see the same variation.
Ultimately, these algorithms can personalize the content of each segment of your audience; however, this method requires a lot of time and many repetitions if it is to succeed.
Whether you use a classic A/B or a bandit test, be sure to follow best testing practices: whether these concern interpreting the data or the moment at which to stop the test.
Learn more about Kameleoon's A/B testing solution which provides a full range of features to let you run simple or complex tests quickly and easily.
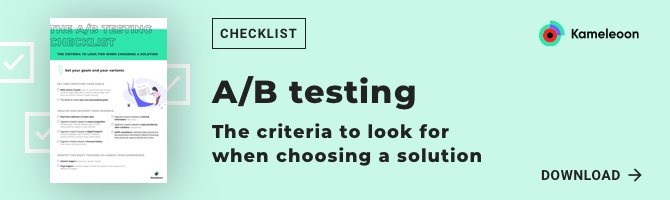