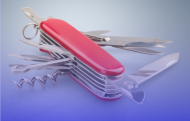
7 experts share their must-have A/B testing tool features
Kameleoon found that 90% of leading organizations combine marketing and product-led growth strategies. And 81% expect significant business growth thanks to their closely aligned tools, data, and processes.
To achieve this, leaders must be intentional about the technology and processes they introduce—and when it comes to A/B testing tools, there’s a lot to consider.
When comparing features, just because something is listed doesn’t mean it’s needed. In fact, too many features make testing tools difficult for less experienced teams to use.
Instead, it’s best to establish the must-have features your teams actually need to “do” testing. Then, evaluate whether those features are built in a way that supports cross-team alignment and collaboration. Consider;
- Visibility. Does the feature help teams know the what, when, where, why, and how other teams work? For example, can marketing see the results of a product messaging test?
- Efficiency. Does the feature make collaboration easy for teams, or does it introduce additional hurdles? For example, are standardized templates available?
- Effectiveness. Does the feature enable teams to centralize or share data? For example, does it allow teams to use data from their specific department tools to enhance customer understanding and improve test segmentation?
Experimentation often begins in one team and then spreads, and each team can require different features to enable them to test. So, what are the must-have features that help all teams experiment? We asked seven leading experimenters to share their list.
1. Shared goals and metrics
Imagine your product team is incentivized to improve customer lifetime value, so they focus on increasing engagement. At the same time, marketing’s goal is to increase traffic.
If these teams can’t see each other's goals, marketing might send more low-quality traffic to the site, which negatively affects engagement rates (and business overall.)
It’s not only the visibility of other team metrics that’s important; as Lydia Ogles explains,
This fosters consistency in decision-making, facilitates comparability of results, optimizes resource allocation, and enhances team collaboration. With a unified approach to measurement, organizations can make informed decisions based on reliable data insights, leading to more efficient experimentation efforts and, ultimately, greater business success.
This standardized approach not only promotes clarity and alignment but also empowers teams to leverage data-driven strategies for enhanced performance and innovation across the organization. By eliminating discrepancies in analysis and promoting a shared understanding of key metrics, teams can streamline their experimentation processes and drive continuous improvement throughout the business.
2. Client-side and server-side capabilities
No matter the team structure (Centre of Excellence, decentralized, etc.) or training, each individual will approach A/B testing with a different level of experience. AI can be your knowledgeable co-pilot, helping to bridge any knowledge gaps.
Help is on hand across the experimentation process, from AI-generated moderated user research scripts to explaining the difference between statistical models and support interpreting test results. Many A/B testing tools natively integrate AI features into their platforms so people can get the help they need without leaving the tool.
The differing experience levels, team makeup, or access to resources will influence how individuals build tests—whether that’s using a self-service graphical interface or coding tests. But it’s not only these differences that dictate what testing tool features are needed.
Where teams plan to test (e.g., website vs. in-product features) also influences whether they need client or server-side capabilities. Shiva Manjunath explains the various features as necessitated by these characteristics,
If they are a low-maturity team with limited resources, I’m a big proponent of WYSIWYG and client-side testing. As long as someone oversees the test development, execution, and launch either through the testing tool or externally.
If you’re a higher maturity team, feature flagging and server-side testing are things you’ll want, to get a more centralized verification of ‘site releases,’ be it “A/B tests” or “feature releases.”
A testing tool that combines both client and server-side testing (a hybrid testing tool) enables teams to conduct experiments using WYSIWYG editors and feature flags.
WYSIWYG graphics editors enable teams to create simple tests without design and developer resources, allowing more teams to run tests and make more informed decisions. But there are clear use cases for feature flags also, as Maya Goradia explains,
While many product teams launch features to a small group of users before general availability, the process doesn't take advantage of the statistical rigor experimentation methods provide.
The additional layer of statistics can isolate signals from the noise and provide confidence in the release.
Experimentation using feature flags can also help estimate the business value of each feature.
It's important to have a feature toggle (kill switch) built into the experimentation tool in case any emergency rollback is needed while releasing new features.
3. Cross-team communication and visibility
As more teams begin to test, there must be visibility of planned and in-motion work to;
- Prevent interference between concurrent tests.
- Refine workloads and maximize efficiency.
- Make the best use of limited traffic.
- Avoid testing ideas that have already been run.
- Enhance the value of each test result by sharing the learning with other teams.
Part of achieving the above is having a centralized and standardized way of running and reporting on experiments. Alexis Grillo shares more detail on what’s needed,
This communication is essential throughout the lifecycle of an experiment, including the details of what is planned, current statuses, results, and recommendations.
This information needs to be readily available to those who are interested in self-serving, or easy to share outside of the tool. It’s all too easy for teams to execute great experiments but then lose efficiency in sharing learnings with a larger audience.
Automated notifications and templates for status changes, results and metrics, and searchable archives are key. Efficiency enables scale, and you want an experimentation tool that can make that happen.
The above mentions an essential point about features that allow you to share information outside of the tool, whether via links to results or reports.
However, test results interpreted by the untrained eye can be misinterpreted. As such, Sundar Swaminathan details the features that help you avoid this when communicating results and decisions,
A.J. Long shares Sundar sentiment about how these features impact an experimenter's mindset,
4. Quality Checks
So much time and effort goes into user research, prioritizing test ideas, designing the experiment, and putting it live. So, waiting for your experiment to conclude only to discover your test results are invalid is a real kicker. Prerit Saxena shares what you need to avoid this situation,
As simple as it sounds, a large percentage of experiments are deemed invalid by the time they end due to incorrect experiment setups. A tool to evaluate the experiment setup comprehensively will avoid quality issues later.
The feature should check for things like power calculation, setting up correct success metrics, trigger setup, demographic targeting, etc. This will improve the quality of experiments overall and make feature teams more efficient.
Shiva Manjunath also suggested that QA features should allow you to preview experiments on top of personalization campaigns. This is particularly useful for understanding how a test will display in a real environment when using complex personalization configurations.
5. Data integrations
Despite companies' best efforts to break down silos, teams often run tests unbeknown to other teams, leading to overlap and redundant results.
Additionally, data held in isolation may not be that valuable. But, when combined with other data, you can:
- Build a rich picture of your customers.
- Conduct advanced segmentation.
- Run AI-driven personalization.
- Model a user's propensity to take specific actions.
For the above reasons, testing tools that offer data integrations are a must. A.J. Long explains,
Integration with these data sources enables all of the teams to have access to comprehensive experiment analysis, empowering them to make informed decisions and conduct more effective experiments.
5 Must-have features for all team experimentation
Here are the top five features needed to support all-team experimentation and gain greater alignment.
Shared goals and metrics.
Ensure cross-team metric sharing for consistent and effective decision-making.
Hybrid testing capabilities to support all-team experimentation.
Look for tools that support both client-side and server-side testing to accommodate various team maturities and testing needs.
Cross-team communication and visibility.
Drive efficiencies by aligning planned and in-motion work. Build trust and avoid biased interpretation with clear visual representations of business impacts and test results.
Quality checks
Use pre-experiment quality checks and visual QA features to prevent invalid setups and improve experiment reliability.
Cross-team data integrations
Check for integration with various data sources to facilitate enhanced customer intelligence, segmentation, personalization, and post-test analysis.
Learn more about how Kameleoon enables all-team experimentation with its hybrid testing tool.
Thanks to all the contributors for sharing their expertise.
- Lydia Ogles, Senior Manager, Web Production and CRO at Vivint
- Shiva Manjunath, Senior Web Product Manager, CRO at Motive and host of the From A to B podcast
- Maya Goradia, Founder at ZD Analytics
- Alexis Grillo, Director Experimentation, Data Science at Albertsons Companies
- Sundar Swaminathan, Analytics Advisor
- Prerit Saxena, Data Scientist II at Microsoft
- A.J. Long, Manager, Product Experimentation & Analytics at FanDuel