What do I need to consider when switching A/B testing tools?
This interview is part of Kameleoon's Expert FAQs series, where we interview leaders in data-driven CX optimization and experimentation. Shiva Manjunath is the Experimentation Manager at Solo Brands. Fanatical about creating high-value user experiences, Shiva has worked on teams spanning B2B (Gartner) and B2C (Norwegian Cruise Line, Edible Arrangements), as well as consulted for businesses working through Speero (by CXL) over the past 10 years. A passionate driver of ‘test to learn,’ he focuses experiments on driving high business value and impact.
How will the sunsetting of Google Optimize affect the experimentation industry?
Firstly, it’s not as problematic as people think. Google Optimize was included for free in the Google Analytics suite of products.
Because of this, it became the default choice for many people. It was a no-friction way for people to start dipping their toes into experimentation and A/B testing, and I am all for that.
There is a significant risk in blindly using WYSIWYG editors to make changes on a site without a proper QA process, for example.
It’s possible many of these folks run tests that actually didn’t make a visual change on the page, then interpret the results as ‘changing the page had no impact’ because it was just an A/A test in reality. Or someone outwardly broke the core functionality of the page, then saw the results tank and interpreted it as ‘change = bad’ when the code just broke the page.
While Google Optimize sunsetting means fewer people will run tests or be exposed to testing, I think overall, it will increase the maturity of experimentation.
Rather than running experiments without proper guardrails and the harm this could entail, companies will be more likely to create a plan, commit budget, and hire staff to run a proper testing program.
While it could be detrimental to experimentation if it turns out businesses needed the free Google Optimize tool to prove it’s worth spending more money on, I’m just not sure this will happen to the level people think it will. You can read more of my musings on this topic here.
What do I need to consider when switching A/B testing tools?
Many people are looking for ‘bang for their buck’ regarding the tech stack, and you can’t really blame them. However, I’d say you should consider the following:
- What is my current tech stack?
- What do I need the most help with?
There are many A/B testing tools out there, all with different offerings. Some specialize in running A/B tests only. Others have a suite of offerings, including heatmaps/session recordings built into the tool. Some are cheap. Some are very, very expensive (but high quality).
So it behooves you to assess where your limitations are. For example;
- Do you already have tools to capture heatmaps/session recordings and run polls? If yes, perhaps you’ll want to focus more on finding a better testing tool.
- Perhaps you have a fantastic dev team and need to enable better and faster server-side testing and don’t care much about a WYSIWYG-type testing tool.
- Perhaps your program is at the maturity level of outgrowing third-party tools. You should consider building your own tools because the cost of a third-party tool working and integrating with your systems is more expensive than developing it in-house.
So, kind of a non-answer because it depends explicitly on your needs internally.
What questions should I ask A/B testing tool vendors?
I’ve outlined some questions you should ask in the pre-sales process to help evaluate and select a new tool. It’s worth remembering that no matter how cool a tool is, if you have a strict budget, you have to purchase a tool within that budget.
- How long will it take from purchase to launching of the first non-A/A test?
- Will the vendor support migration of segments/data/past tests into the new platform (if you have an existing tool)?
- How scalable is the platform/pricing model?
- Can you run unlimited tests with unlimited impressions, or is there a cap?
- If there is a cap, what is the process/price for scaling beyond that cap?
- Do you get a discount if you bundle the testing tool with other tools they might offer?
- What is the cost in terms of users? Think about how many developers may need access to the tool.
- Do users have unlimited access?
- What does support look like if I need help with my experiment setup/test results/data etc.?
- Does the tool allow for deep dives into the data/analysis WITHIN the tool? Or does the data need to be exported/pushed into a data lake/analytics tool for further analysis?
- Are there any significant impacts on site speed associated with how the tool will be implemented?
- What does project management look like in the tool?
- Is the dashboard customizable/helpful to you?
- Does the testing tool integrate well with other project management tools such as Airtable, Jira, etc.?
- Provide information on your tech stack and see if the tool will work well with it.
- Bonus: have your engineering team look at the technical documentation for the testing tool to ensure it will also work well with the site’s tech stack.
- Bonus: have your engineering team look at the technical documentation for the testing tool to ensure it will also work well with the site’s tech stack.
What criteria should I use to evaluate and select a new A/B testing tool?
Here is a quick (non-comprehensive) list of things to consider or decide on. Once you have the answers, you can create your evaluation criteria which set out what the new testing tool must have/do.
- What is your budget?
- Do you need server-side testing or do you primarily run client-side tests?
- Do you need heatmaps/session recordings?
- Do you need Multi-Armed Bandit testing? Personalization? Feature flagging?
- Do you need in-app testing?
- Do you have a complicated tech stack where integrations may be harder with the tools you have?
- How advanced are your data scientists?
- Does the tool have built-in data accuracy features like an SRM checker?
- Are you using the tool to help understand if things are statistically significant?
What operational elements are key to making the switch as easy as possible?
Again, a lot of your Google Optimize migration will depend on your specific business, processes, and tools. But one element that is key to making all switches go smoothly is data quality.
To make sure this is the case:
- Consider how you maintain consistency across records.
- Run an A/A test after everything is set up to ensure data is reporting accurately.
- Check to ensure all the targeting works as you need it to.
You should also use this time as an opportunity to audit your experimentation process/program management as a whole. Are there things you should change but haven’t had a chance to? Make those changes now while the metaphorical ‘patient’ is open.
We love your pooch, Jordy. Have you learned any lessons from dog training that you apply to your work?
Always test things out before you fully commit to them. Otherwise, you will spend your money on a bed that everyone says dogs love and Jordy will sleep next to but not in. It turns out he loves another style of bed more than this…lesson learned.
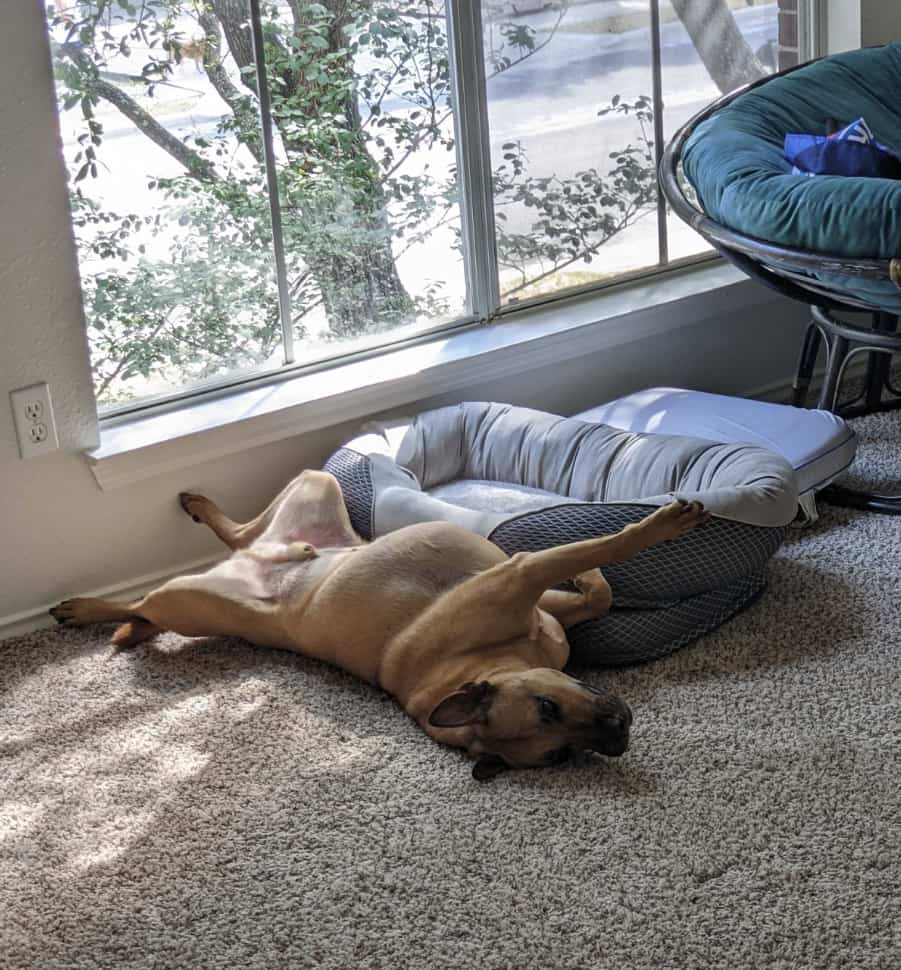