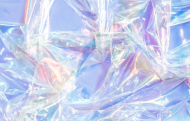
Can you really tie A/B testing to revenue?
Expressing the ROI of A/B testing, especially in terms of revenue, is far more complex than most brands (and optimizers) realize.
This is how the sales pitch usually goes: CRO agency, tool, or consultant says, “Our test generated X more incremental revenue. If we roll out this test to 100% of traffic for the full year, you’ll generate [enter big $ number].”
Sounds familiar right?
When summarized like above, the proposition is absurd to most optimizers. Expressing the ROI of A/B testing, especially in terms of revenue, is far more complex than most brands (and optimizers) realize.
Yet, that’s precisely how the value of a/b testing and experimentation is often sold, even by highly established players in the industry.
So, can you accurately extrapolate a winning experiment's results for revenue forecasting?
Can the results of a fully-controlled experiment hold for a full 52 weeks?
And, if not revenue, then how the hell else does one communicate the value of experimentation?
We invited David Mannheim, global VP of experimentation at Brainlab, Ben Labay, Managing Director @ Speero, Craig Sullivan (CEO @ Optimise or Die), and Chad Sanderson (Head of Product, Data Platform @ Convoy) to a "Kameleoon Live Session" to figure out how.
Experimentation is more about making informed business decisions, which gives managers and companies a far greater chance of growth and profit than puffing up vanity CRO metrics. Remember these mantras to remind yourself of your value as an optimizer.
- Be the laboratory, not the test.
- Be the compass, not the map.
- Sell the insight, not the widget.
We’ve summarized more key insights and takeaways from the live debate and discussion below.
More people disagree that you can link an a/b test to revenue than not.
David Mannheim's article series, Why do we assume experimentation is linked to revenue? and poll generated a swarm of comments and hand wringing when posted. We called it his Jerry Maguire moment.
Most people agreed with him! Calling a/b testing out is akin to having the courage to say the emperor isn't wearing any clothes.
Most companies want shortcuts to a pot of gold.
David is not alone in his frustration with the "over-indexing of a/b testing to revenue" (Everyone blames (and credits) Optimizely, FYI.) Like anything worthwhile, delivering value through experimentation takes work. Lots of it.
Oliver Palmer echoed David's sentiment in his blog article, "The crisis of optimisation." He writes:
"[Experimentation] is not a lottery ticket, but rather an opportunity to observe and measure reality. Most clients don’t want reality, though. They want wins. And if agencies don’t deliver rivers of gold (or claim to have done so), they can expect to lose that client to the next contender who promises that they will. Internal teams that don’t claim significant uplifts are at risk of losing funding, credibility, prestige, and even their jobs."
Don’t make promises you can’t keep.
Don't oversimplify an experiment's ROI calculation. Or, don’t multiply by 52. Limited understanding of calculating an experiment's "annual" business or revenue impact has people multiplying their experiments' observations/conclusions by the number of weeks in a year. This math doesn't work.
Back in the nascent CRO industry days, Craig thought like most people: "This thing will pay off for another 52 weeks. I just need to like multiply it by 52, and it's fine, right?"
But his understanding of how an experiment's ROI is calculated evolved with time: "Then I realized… that value is actually a range," and that the actual number could be higher or lower than that. "There's a great deal of uncertainty."
You’re not a crystal ball. You’re a compass. At one point, projections like these started feeling utterly arbitrary. "See, that value I'm thinking about happening in the future is completely illusory." "You don't even know if the thing that you ran last year will still work if you run it today," because markets change, competitions change, budgets and prices change along with other internal and external factors. Things, in general, will not be the "same at that future point as they are now when you run the experiment and get the data."
Craig suggests placing more care on finding forward momentum — that's the real purpose of experimentation.
Tie experiments to goals and insights; avoid extrapolating that a test will result in $X.
Experimentation is about making more informed decisions and not necessarily about making more profits.
Don’t set yourself up for failure. Experiments don't say "anything about what's going to happen in a month or what's going to happen in six months or what's going to happen in a year," says Chad. Recalling a two-week experiment he conducted on Subway's website, Chad shares how the test that originally resulted in a 3% lift produced nothing of significance when it was rerun a few weeks later. "We saw no result there; there was nothing." If he had gone around the office touting the ROI based on that test, it would have led to disappointment and confusion about the value of his optimization program.
Experiments are about separating signal from noise and better decision-making. Knowing what Chad knows today about experimentation, he'd go so far as to "eliminate" his old job at Subway! Chad stresses that experiments should be a part of the infrastructure and be seen as "a framework for enabling decision-making across the company."
OKRs can help with setting meaningful goals for experimentation. Chad goes on to explain how at Convoy, OKRs (objectives and key results) guide experimentation. "A lot of our OKRs are based around experimentation results, so if you want to say we have an OKR around improving margin by 5% or 10% over the course of the quarter, usually there's another sentence that says as proven by experimentation added on to the end of that OKR and that creates a really interesting incentive structure in the organization where now people are thinking about when you're building features, how do we incorporate experimentation so that we can attribute as much of this margin gain as we can to the efforts that we're implementing."
Experimentation is as much about maximizing rewards (profits) as it is about risk aversion and mitigation.
Ask, what’s the cost of NOT acting? With experimentation, companies get to make informed decisions and bypass the risk of not acting. Experimentation can guide data-driven decisions -- launching freemium plans or free trials, for instance. There's a lot of exploration to do here, and experiments are the key.
Selling such risk aversion/mitigation benefits of experimentation to stakeholders in marketing-led organizations, though, is more challenging as pitches promising rewards and making more money are more appealing.
Tie revenue to experiments — you have to — but be wary of transferability issues
The CRO industry is built on the idea of tying efforts to revenue. It lives in the marketing/business world, which is revenue-driven.
"Everything points back to revenue.” It's the ultimate measurement system in the business world," says Ben. So you "have to attempt to attribute revenue to experimentation and your efforts." Clients at Speero are actually asked for data that helps the agency create measurement systems to help with business i.e. revenue-affecting, decision-making with their experimentation.
Experiments' results don’t guarantee long-term projections. But revenue measurement shouldn't include loosely based long-term projections, cautions Ben. "We only tie it to revenue within the statistical model of the test; we don't try to project out… and that's where you get into trouble when you're trying to measure the ROI of a program based on a set of test statistics that are not meant to be transferable in space or time." Your results from experiments, including movement in core revenue metrics, should lead to better decision-making — that's the idea.
Know your audience, as marketing-led and product-led organizations see things often VERY differently
In marketing-led organizations, a/b testing is about gains and margins. Here, optimization is about "finding local maximums or trying to shake yourself out of local maximums to find some new ones," explains Ben. Helping them find ways to save on acquisition costs and/or boost retention - speaking in terms of revenue metrics makes sense.
Product-led organizations use experimentation primarily to learn. This other side of the spectrum uses experiments more for innovating and transforming than generating revenue. These businesses want to grow through innovation and be truly different and better than the competition. Experimentation is viewed differently in this environment and is about directing and investing engineering or resources in the right places. While they do translate to revenue, they aren't pegged at a dollar value.
The shift (marketing-led→product-led) can happen. Optimizers can try to shift the ROI conversations in marketing-led organizations by making them see the value of insights that experiments generate and how they tie to their growth goals. Think of goals like more simplified and personalized shopping experiences instead of an X% lift in conversion rates. Another way Ben suggests is translating growth strategies for such businesses that qualify as solid hypotheses and running experiments on those.
Don’t kid yourself. But turn the tables.
Experimentation lives in the real world, where many senior stakeholders believe revenue metrics are the end all be all. Fine. They’re not wrong, but directly correlating a single a/b test to revenue more likely is. Consider pitching the value proposition of your optimization program based on the insight it delivers. When you learn something new about “their” customers ask, “Based on what we’ve learned from this experiment, how can I help you make a business decision?”
Want more?
Helpful reading. Here are some experimentation blogs and links recommended during the Live Session. Craig recommends, Why we use experimentation quality as the main KPI.
- Booking: booking.ai,
- LinkedIn: engineering.linkedin.com/blog/topic/experimentation
- Netflix: netflixtechblog.com/tagged/experimentation
- Airbnb: medium.com/airbnb-engineering
- Spotify: engineering.atspotify.com
- Twitter: blog.twitter.com/engineering/en_us.html
- Pinterest: medium.com/pinterest-engineering
If you registered, listen to the replay. We only scratched the surface in this recap. LOTS more amazing insights and “uh-huh” moments to listen to (and watch) in the Live Session.
-
What do you think about linking experimentation to revenue? Did we miss something? Didn't register, but want to see the replay? Let us know? Write to us at [email protected]. A big thank you to Ben, Craig, Chad, and David for having this much-needed conversation!