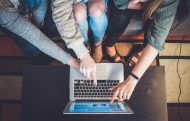
Manual or predictive on-site personalization – what is better?
According to research, 4 out of 5 marketers believe that personalization is essential to successfully compete today. Therefore, companies are gradually abandoning mass-marketing strategies—i.e. one message for everyone—in favor of increasingly accurate customer segmentation. This allows them to be able to personalize customer experiences, offers and messages.
When it comes to personalizing on-site marketing actions there are two methods of achieving this:
- Manual personalization (governed by fixed, pre-set rules),
- Predictive personalization (based on flexible, machine learning algorithms)
What are the differences between the two and which is best suited to your business challenges? Are they exclusive or complementary? This article aims to provide the answers, illustrated with relevant examples.
1 What is personalization?
Personalization involves adapting an offer, message or content to an individual target. So before brands can personalize, they need to know who they are addressing. Whether it’s manual or predictive, personalization relies on the collection and processing of visitor data to identify the needs of a target before offering them a relevant browsing experience or specific marketing action.
1. MANUAL PERSONALIZATION
With manual personalization, the targeting and triggering of your contextualized actions depends on rules (or criteria) that have been decided and set in advance.
Segmenting is performed based on the real-time analysis of so-called “hot” data on the one hand—i.e. the data linked directly to the context of the visit in progress on the website (behavioral, contextual and technical)—and of “cold” data on the other (i.e. historic visitor data from your CRM, DMP, CDP, etc.).
Once segmentation is complete brands can push on-site marketing actions to the different visitor segments of a website (for example, new visitors, customers for more than two years, customers using mobiles, cart abandoners, etc.).
2. PREDICTIVE PERSONALIZATION
As for predictive personalization, it doesn’t depend on rules that are static, but is based on real-time analysis of all visitor data by artificial intelligence. This type of personalization relies on machine learning algorithms.
Machine learning is a branch of artificial intelligence which consists in feeding data to an algorithm, which then learns from it to make predictions.
There are three key stages in its use:
1. Assign the algorithm a goal
The role of the algorithm is to measure the conversion probability of each visitor to your website in real-time. The first step is therefore to establish which “conversion” is required. The conversion goal given to the algorithm can, for example, be the purchasing probability in a given price category, or even the probability that message A will convert a visitor more effectively than message B.
2. The algorithm feeds on all visitor data within the data ecosystem
The algorithm begins its learning process using the hot and/or cold data that it is fed. It learns from the behavior of the visitors to the website, searches for correlations between visitors and improves its capacity to determine each visitor’s conversion probability over time.
3. The algorithm identifies in real-time each visitor’s interest in specific marketing actions
The algorithm determines each visitor’s conversion probability and adjusts its actions accordingly (triggering contextual actions only when relevant).
« It’s not enough to ‘plug’ an algorithm into your website and cross your fingers for it to automatically identify correlations between visitors. The marketer must first assign it a clear goal and configure it so that it prioritizes processing the data that has an influence on the given goal. The algorithm improves its predictions in real-time and identifies the optimal target for your message or offer.»
Abdel El Ouazzani, AI Consultant, Kameleoon.
HOW DATA SCIENCE EVOLVED FROM A MANUAL TO MACHINE-LEARNING MODEL
In the field of data analysis and processing, rule-based manual personalization systems work flawlessly, provided that all eventualities are known in advance by the person who sets the rules. In the past, smaller traffic volumes, less complex data and more standard customer journeys made the setting of these rules relatively simple. Today, with high volumes of complex and at times unstructured data, it is increasingly difficult to stick to a rule-based system. This is where machine learning comes in.
If we classify any kind of data by categories (A, B, C, D, E, etc.), a machine learning algorithm can learn, by itself, what defines the elements of each category and process this data in real-time. This evolving model is taking the lead in data science, but also in marketing and personalization.
2 3 examples to compare manual and predictive personalization
1. FROM A “1-TO-FEW” APPROACH TO A 1-TO-1 APPROACH
From a “1-to-few” approach...
Manual personalization is a good option if a brand has a specific idea of the customer segment it wishes to reach and the criteria that define it: “I want to reward loyal customers who have spent more than €200 over the past six months, by inviting them to an event”; “I want to push a welcome offer to my new visitors”, etc.
For example, the team at French electrical retailer Darty can configure Kameleoon to offer:
- Free delivery to all new visitors arriving from a SEA (Search Engine Advertising) campaign and who are visiting the website from abroad (WHO).
- A free delivery code, via a pop-in (WHAT)
To define the target of this personalized campaign, they would therefore use the following criteria:
- Visit number (first)
- Source (SEM)
- Geolocation (Outside of France)
The targeting (WHO) is therefore established according to manually set rules. As for the action that follows (WHAT), this is also prepared in advance: the marketing team decides which action(s) they wish to trigger and prepares the design of the pop-in and the conditions in which it is triggered in advance.
…to a 1-to-1 approach
With predictive personalization, brands go beyond the notion of segments, letting the algorithm determine the optimal target for its action based on the characteristics of each individual visitor.
For each visitor, the algorithm calculates a conversion probability at a given moment. Based on this probability, it triggers—or doesn’t trigger—a relevant marketing action that it can contextualize in real-time. The following graph shows the distribution of visitors to a website according to their conversion probability.
Based on this, a marketing team can decide to trigger a promotional action just for “warm” customers, to maximize the chances of them buying. It’s pointless to do this for those whose purchasing probability is high and who would convert anyway (as this would impact margins) or low (as this would spoil the customer experience as they have no or little chance of purchasing). On-site marketing actions are thus individually targeted rather than being targeted by segment.
Essentially the predictive algorithm is necessary when it’s not easy to precisely identify a target because the criteria that define it are vague, ambiguous or shifting: “I want to push a discount coupon to undecided visitors”, “I want to target visitors influenced by the scarcity effect to push an urgency message”, etc.
One example where this has delivered benefits is Toyota, which moved from manual to predictive scoring to identify potential car buyers and encourage them to visit their closest dealership for a test drive. The aim of the Toyota website is not to push online car sales. Instead, it tries to generate qualified traffic for its physical dealerships - it aims to ensure that only potential buyers are being sent from the Toyota website to dealerships for test drives.
The main priority is to avoid offering test drive to visitors with no or a very low purchasing intention, since the process is costly and time-consuming. The goal of Toyota.fr is therefore to identify as accurately as possible those visitors who are showing a real interest in purchasing a car. They then receive a significant offer: the chance to test drive a vehicle for several days.
Pop-in presented to potential buyers to request a test drive
At first, Toyota used manual scoring to define its “potential buyer” target visitors. A score was given to every action the visitor performed on the website, such as viewing the Toyota vehicle configuration page, time spent on a vehicle page, total length of time on the website. As soon as the visitor exceeded a certain score, the test drive pop-in was triggered. The result was a marginal improvement in quality of leads.
This demonstrates that manual scoring provides an imperfect approach to what defines a potential buyer. It proves that defining “a potential buyer” is instead based on more ambiguous and shifting criteria that are mainly linked to behavior on the website and the context of the visit.
To overcome its challenges Toyota adopted Kameleoon’s AI to identify the purchasing interest of website visitors. This intent is determined by analyzing the large quantity of data relating to visitor behavior and to the context of the visit. By processing this data 24/7 and adapting to how visitor behavior evolves, the algorithm is logically much more relevant than a static human approach. The number of “qualified” leads that turn out to be potential buyers of a Toyota vehicle has thus doubled. The predictive approach enables Toyota to considerably reduce the number of test drives by those that ultimately have no intention of buying.
With Kameleoon’s AI, Toyota also personalizes the test drive request pop-in by adding the name of the model for which the visitor is showing interest along with the location of their nearest dealership.
« We needed a solution capable of calculating our visitors’ interest in the Toyota brand and our car models. Manual scoring yielded an initial improvement in the quality of the leads sent to our dealerships, but it was with Kameleoon’s AI that we considerably improved the ratio of purchases/to test drives. Our dealerships are grateful.».
Julien Descombes, Head of Digital Communication, Toyota
2. STATIC SEGMENTATION VS. DYNAMIC SEGMENTATION
Manual personalization is not dynamic: the rules are established from the outset and only evolve if someone changes them. Looking at the example of Darty shows that manual personalization doesn’t “discriminate”. An identical experience is offered to all the visitors who meet the criteria for belonging to a specific segment (in this case new visitors coming from an SEM campaign).
Conversely, predictive personalization is dynamic and predictions evolve over time along with the behavior of website visitors. The algorithm learns and refines its targeting criteria in real time to only trigger a contextualized action when relevant (i.e. when it determines that the conversion probability of a visitor requires an action to be triggered). The animation below shows how the algorithm learns, week after week, in the case of a predictive use case set up for French online tire retailer Allopneus.
3. THE VOLUME OF DATA NECESSARY TO ADOPT PREDICTIVE PERSONALIZATION
One of the questions most frequently asked about the predictive approach is around the volume of data necessary for it to be effective. Contrary to popular misconceptions it doesn’t necessarily need a high volume of data. However, it will logically require time to train the algorithm.
More importantly, a low volume of data to process can implicitly indicate a low potential for improving conversions, whether this means increasing turnover or margins. The “risk” is therefore that the marginal gain afforded by the predictive algorithm will not be very significant. It’s also worth assessing what is hidden behind the notion of data “volume”. If it’s just a large quantity of easily identifiable segmentation data (see above), then manual personalization will be just as effective. But if by “volume” we mean a large quantity of disparate data which helps identify a target, then the predictive approach will still be necessary. This is because the algorithm will be much more efficient at sifting through this varied information and picking out the criteria that are the most relevant for the required action.
Let’s take the example of an e-commerce player wishing to win back cart abandoners with a promotional offer (free shipping). With manual segmentation, the retailer creates an “abandoner” visitor segment by cross-referencing two criteria:
- The cart is not empty
- The visitor’s mouse has left the website area.
A “Free shipping from $x spent” pop-in is triggered to try to retain this segment. Since this doesn’t require much visitor data, the personalization action is easy to undertake. With a predictive approach, the algorithm identifies abandoners. It will feed on much more data than the two criteria listed above. The algorithm will identify many more abandoners by including those who have taken no action for x seconds or are loitering on other pages, opening other tabs, etc.
Additionally, it will perhaps weed out some of those leaving the website, because their conversion probability will be deemed low. In theory, the predictive approach will be more accurate and it will perform better than the manual model. What remains to be seen now is the gain generated. If the website traffic is low, there will certainly be a gain but it could turn out to be totally marginal. The higher the traffic, the higher the potential gain.
In a nutshell, with low traffic and very little data to feed into the algorithm, predictive personalization is entirely possible, but the gain may be marginal. On the other hand, if you have sustained traffic and a large volume of data to work with, then the predictive approach will be a force to be reckoned with.
«The more visitor data the algorithm has to analyze and learn from, the faster its learning will be.» -Ronan Sy, Full Stack Engineer, Kameleoon.
3 Manual personalization vs. predictive personalization: should you favor one over the other?
Given that the algorithm improves and adapts its prediction capability in real-time and 24/7, its performance is, in theory, always better than a human’s performance. This is particularly true if the hot data (behavioral or contextual visit data) plays a decisive role in identifying the optimal target for your actions.
Many studies, such as the one conducted by Benjamin Shiller, demonstrate that this data is by far the most effective for determining a visitor’s interest in an offer. If this is your goal, then the predictive algorithm is necessary.
However, this doesn’t rule out the manual approach for actions where the target has been clearly identified and has easily configurable characteristics (new vs old visitors, desktop vs mobile, etc.). However, bear in mind that with the growing number of personalization actions being undertaken on a website, the manual approach can turn out to be painstaking and hard to orchestrate.
One of the clear advantages of the predictive approach is also that it enables you to automate actions and it can better manage data volume, complexity and variety compared to the human brain.
If you’re looking to maximize the ROI of your on-site marketing actions, contact our team. They will be happy to answer all of your questions and help you achieve your aims.