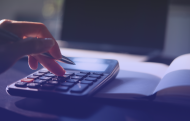
How to evaluate the ROI of your testing program
A testing program's return on investment (ROI) is a key talking point at two milestones.
The first is when business leaders are asked to invest in A/B testing tools or hire dedicated staff for the first time. The second is when the program scales and needs advanced evaluation methods to capture the real value of experimentation.
ROI calculations tally revenue uplifts from experiments against costs. This calculation is an adequate way to evaluate initial investments.
However, as experimentation programs mature and measurement improves, more sophisticated analyses attempt to qualify experimentation's broader impact. This might include measuring opportunity costs, savings, customer lifetime value, innovation, and long-term value creation.
The ability to quantify the value of experimentation is essential for senior professionals—not just for justifying investments to stakeholders, but for refining methodologies, optimizing resource allocation, and driving continuous improvements. This article explores how eight experimentation leaders evaluate the value and ROI of experimentation and offers advice for leaders who face similar challenges in their organizations.
Measuring experimentation ROI
At the start of any new testing program, ROI will be essential in securing buy-in and investment. But what factors should you include in your calculation? Jess Vandenbruggen breaks it down:
Validated value is the revenue improvements validated through experiments. Opportunity cost is the revenue loss from experiments that, in a world without testing, would’ve been in the ‘just do it’ pile.
Being transparent about these value propositions and the investment of time, teams, and tools are key to showcasing the value of experiment programs and gaining further business buy-in.
The simplicity of the ROI calculation can lead to issues. Different interpretations of what constitutes a “cost” and “return” is a common pitfall. Spencer Whiting shares some other commonly overlooked factors when calculating the ROI of your testing program and how you should overcome them:
To do this, you need to track all associated costs, including tools, labor, and additional expenses. Key factors include incremental revenue, conversion rate improvements, and long-term impacts like customer retention. Determining attribution parameters before you start is important to have a clear picture of the true ROI of your experimentation program.
Commonly overlooked factors include the long-term value of improvements, qualitative user feedback, and the time and resources spent on analysis and implementation. By considering these elements, companies can accurately assess the value of their experimentation efforts.
The limitations of using ROI for experimentation
One of the biggest issues with ROI calculations is there’s no defined timeframe in which you measure costs and returns. Are you including expected annual returns or counting only realized revenue increases? Whatever you decide, keep it consistent, and make sure it’s clear to anyone using the figures.
The other issue with ROI is that it only measures financial returns. But the value created by experimentation goes way beyond revenue.
Revenue targets can also become perverse incentives that encourage the wrong behavior. Suppose an experimentation program is solely measured on revenue uplift. The costs associated with increased product returns might not be factored into ROI, obscuring the real impact on the business.
Maria Luiza de Lange recommends setting out key metrics early on to avoid this:
This can be achieved by establishing North Star metrics, collectively known as the Overall Evaluation Criteria (OEC). These metrics might include growth indicators, such as the additional revenue generated by an improved site version or the revenue preserved by avoiding the launch of a poorly performing feature.
The OEC can also encompass cost-saving measures, like reducing customer support calls due to improved online content. By setting an OEC baseline and leveraging metadata, you can establish clear targets and enhance the value that A/B testing contributes to the business.
The value of cross-team collaboration in A/B testing
The value created by experimentation can be wide-ranging, and the factors that support it can be hard to quantify.
Take user research. The outcome of tests derived from user insights might have a higher test success rate. However, attributing specific user research costs to specific tests isn’t easy.
It’s also hard to quantify the impact of cross-team collaboration on experimentation. However, Kameleoon's 2023 Experimentation and Growth Survey found that 75% of high-growth companies have an experimentation program that supports cross-team collaboration.
We also know why this type of cross-team collaboration is important in testing:
Alternative experiences, different cultures and heritage, and perspectives that vary from interests, mindsets, and ideologies can breed fantastically diverse thinking.
Involving teams across your business in experimentation to increase the value of, and impact on, experimentation is a great idea. But there’s a barrier to measuring the costs and value of such initiatives, as Chris Gibbins explains:
However, this only really works for centralized teams in total control of the end-to-end process, from research and ideation to development and analysis.
In companies where A/B testing is part of numerous roles, calculating the costs and returns becomes tough. But there are ways, as Chris explains:
The reality, though, is that when most organizations become mature enough to have embedded experimentation practices in this way, they’ve usually moved beyond having to justify the existence of the Experimentation Team, and there is less of a need to report on the ROI of their function. They are now an essential function for the organization.
The true value of experimentation
As teams mature in their use of experimentation, they move away from basic UX testing towards informing business decisions, including pricing, strategic direction, and USPs. To do this, an internal culture must be built around data and insights rather than gut instinct.
But how can you measure the value of an experimentation culture?
Manjot Jaswal shares how she does it at the RS group:
-Velocity – this tells you how fast you are gaining valuable insights
-Conclusive rate - including the wins and the losses. This helps measure the quality of your experiments.
-Adoption rate – how many teams have started their experimentation journey and are growing their experimentation mindset?
Experimentation is a true team sport. Therefore, cross-team collaboration is a powerful way to supercharge your testing program and increase the above.
At RS Group, we trialed having a dedicated, multidisciplinary squad focused on experimentation. This quadrupled the team’s velocity via smoother processes, increased our conclusive rate due to triangulating data points gathered from multiple teams, and greatly impacted revenue.
Scaling the value of your experimentation program
Cost-related decisions and the performance of your tests will impact the point at which your testing program generates a positive ROI. But these are constantly changing figures.
As your experimentation program grows, the team structure (CoE versus decentralized) will impact the ongoing costs for training and staffing.
Likewise, using off-the-shelf A/B testing tools that scale and integrate time-saving AI features would reduce outgoing costs. This is compared to the cost of building an in-house tool, with ongoing development and hosting costs.
Whatever the target is for ROI, as time goes on, you’ll want to show that your program continues to generate results. Here are four ideas to help you improve your performance.
- Track program metrics and OECs, such as the velocity of tests and ideas implemented. Review these metrics regularly and identify any factors that limit them.
Scale testing efforts across the company. “Prioritize optimizations that offer potential for scaling - at a template or audience level to maximize impact.
"We start with a swimlane analysis to ensure we're prioritizing tests based on what will deliver the highest incremental conversions, based on evaluating traffic and conversion volumes, and projected conversion lift based on qualitative and quantitative analysis.” Laura McGuire, Group Director of CRO at Dentsu Media.
- Use AI tools or features to save time and improve the quality of your work, from testing analysis to reporting: “When applied thoughtfully, AI can supplement every single stage of the experiment production process to either save time or increase quality (or ideally, both).” Mike St Laurent, Managing Director, NA at Conversion.
- Effectively communicate. “Have a clear communication strategy for primary and senior stakeholders and the wider business to increase adoption and build buzz. Be it newsletters, forums, or tailored training sessions, be sure to share exciting experiment results, process improvements, and ways of working.” Manjot Jaswal, Experimentation Specialist at RS Group.
Thanks to all the experimentation experts who contributed to this article.
- Jess Vandenbruggen, Head of Digital Experience at Drumline Digital
- Spencer Whiting, Senior Conversion Marketer and Principal at Whiting and Company
- Maria Luiza de Lange, Head of CRO at Leovegas
- Chris Gibbins, Chief Experience Officer at Creative CX
- Manjot Jaswal, Experimentation Specialist at RS Group
- Mike St. Laurent, Managing Director, NA at Conversion
- Laura McGuire, Group Director of CRO at Dentsu Media
- Dan Truman, Director at Duga Digital